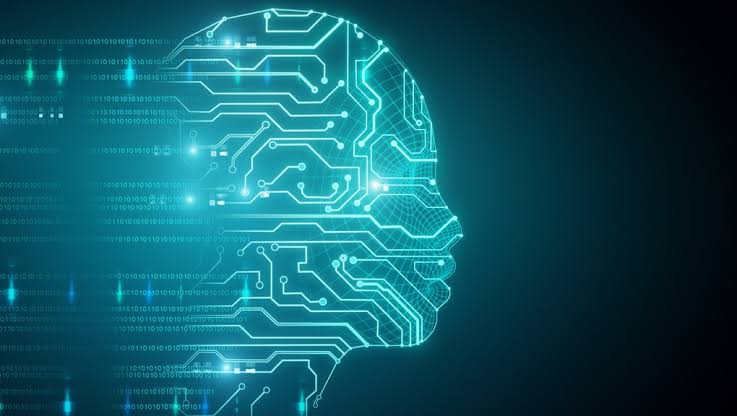
What is AI?
Natural Language = NLU (awareness), NLG (generation), text mining (existing)
Robotics = Driverless Cars and Automation (New)
Computer Awareness = Visual Recognition (New)
Machine learning (ML) = deep learning (DL), reinforcement learning (new), supervised and unsupervised (existing)
Expert Systems = Knowledge Engineering (New), Business Rules (Existing)
He was surprised by Bank of America's "Erica" Virtual Financial Assistant
Bills paid anywhere, anytime
Sent money to friends
Spoke, typed and pressed
"Is the data extracted from this app and the data included in the models they are building along with other customer interaction data?" He has a great question for BoA data analysts.
Artificial Intelligence is a Traditional text mining text captures context from the text that comes into attendance pattern - it has been around for over 20 years.
USM’s AI services help you automate your entire work environment. Know How?
Driverless cars run through the practice of reinforcement and people say, "The model is not great now, but we learn from its mistakes." However, supervised ML drives most of what we do but it declines over time.
Visual recognition is making huge profits and the speech is driven by DL, which is different from ML. DL requires different raw materials and greater volume. It's not like Churn Models and Predictive Maintenance. DL focuses on speech, text, and visuals.
What’s different is that all this is happening without programming. The nervous nets fell asleep. They are becoming more and more popular today.
DL is exciting in what it can do but needs a new focus.
Samples with ML are generated by the algorithm. Why is the phrase "cognitive" so hot right now?
ML is a broad term that usually refers to the careful presentation of curated data to computer algorithms, which find patterns and generate samples systematically (formulas and rules). Although the algorithms are explicitly programmed, the models are not.
Carefully curated data is sent to the algorithm built by man.
The monitored ML is given a dataset with "target variables" and "input variables". The modeling algorithm automatically generates a model (formula or ruleset) that makes a connection between the target and some or all of the input variables. There are many algorithms anywhere from one to a use case.
The 1,000 driverless cars are all hooked up so learning from one can be conveyed to others.
If we have three years of fraud data, all of this must be submitted to the algorithm. However, we must remember, when doing supervised practice with historical data, it can be degraded.
With binary communication, everything becomes a supervised practice because you want to make good decisions. It is difficult to come up with an intervention strategy for the next medical diagnostic code against admission / readmit in health care. That is why the definition of the problem is important. The black box model may not be appropriate because a simple decision tree or regression is good.
What then is unsupervised learning? Having a goal is not enough. When the whole model does not apply to what is right or wrong, it is not monitored.
All unsupervised practice is to find natural groups and determine if they are common or rare.
See Malcolm Gladwell Teddock on Spaghetti Sauce. More than a third of Americans prefer vegetable slices in their spaghetti sauce. They found something they were not looking for.
Are computers “teaching themselves?” The Google brain went from “diagonal line mode” to “cat mode” to “face mode”. The data is becoming more granular.
If I have three billion transactions, should I sample a few transactions? How can you randomly choose if you don't know what transactions they belong to? Active customers from the previous year? You can't get into too many rows; However, you usually run very short.
Deep learning tells different stories with tens or hundreds of millions of data.
All roads lead to binary classification. Most solutions will eventually be used as classification models.
Real-world deployed solutions are not a model, they are a series of models.
Predictive Analytics is the selection and analysis of historical data, which is collected at the usual time of doing business. Predictive models are built by finding and validating previously unknown models, implementing models, and scoring current data.
Now HR and middle managers are emphasizing programming with HR, Python, and other tools.
How do we use point-of-sale (POS), healthcare and transactional data that are not precisely defined? We have to be careful about what data is relevant or applicable. If there are unregistered cases (i.e. death) when closing, there is no need for more. Symptoms need to be dynamic in nature.
Translate Predictive Analytics into Problem. Very Predictive You need an acceptable view to run the model. Start with acceptable deployment scenarios. If you have insights on hand, that's great.
Data -> Models -> Scores = Goals
Find out how you're going to use your results upfront.
Be aware that the minimum number of records to score is one.
Model building is not computationally complex.
How often you run a model depends on how many variables are changing.
Decisions are driven by data and scores, equations and rule sets.
PMML is a predictive modeling markup language and it has been around for 20 years. Most languages are PMML compatible.
One of the first major uses of landline churn data mining was in the 1980s.
Once the model is built, what is the process like a scientific method? There is no statement of the hypothesis. There are mostly yes / no questions in statistics. Revisit the KPI of the business that originally led the project.