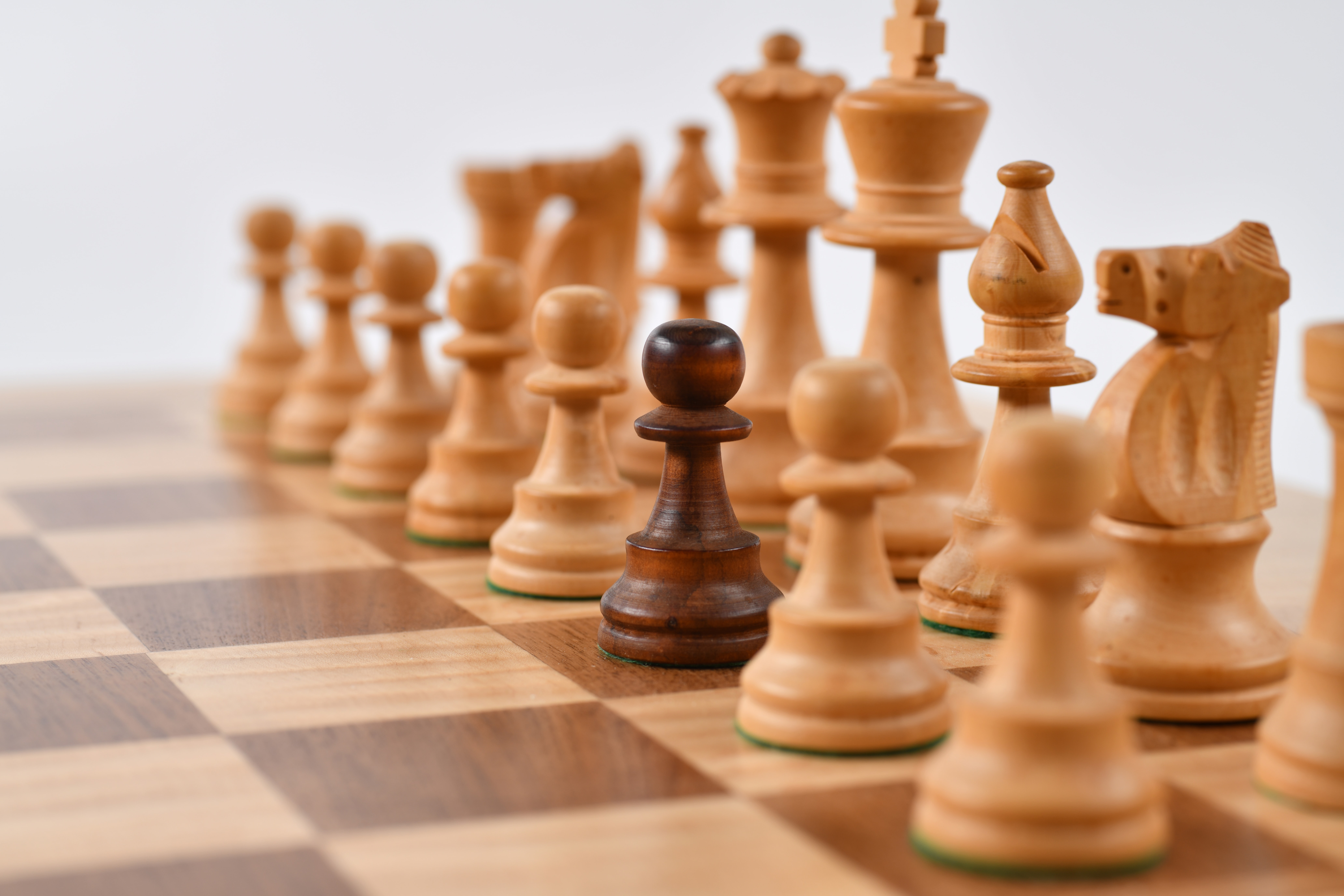
The discovery of unexpected occurrences, observations, or things that deviate considerably from the norm is known as anomaly detection, also known as outlier detection. Any sort of anomaly detection, which is frequently applied to unlabelled data by data scientists in a process known as unsupervised anomaly detection, is based on two basic assumptions:
- Anomalies in data security are quite infrequent.
- The characteristics of data anomalies differ greatly from those of regular occurrences.
- Anomaly data is usually associated with a problem or an uncommon event, such as hacking, bank fraud, malfunctioning equipment, structural faults / infrastructural breakdowns, or typographical errors.
What is Anomaly Detection and How Does It Work?
Anomaly detection is the process of identifying unusual occurrences, things, or observations that are unusual in comparison to conventional behaviours or patterns. You can know more about them with the information security courses online.
Interesting incidents are not uncommon in the area of network anomaly detection/network intrusion and abuse detection. Unexpected spikes in activity, for example, are usually noticeable, even if they fall beyond the scope of many classic statistical anomaly detection tools.
What Are the Different Types of Anomalies?
Anomalies can be categorised in numerous ways:
Anomalies in network activity are deviations from the norm, standard, or anticipated behaviour. Network owners must have a concept of expected or typical behaviour in order to discover network abnormalities. The detection of abnormalities in network behaviour necessitates ongoing network monitoring for unusual patterns or events. The cyber security PG course will help you get the details in the best manner.
Abnormalities in application performance: These are anomalies discovered by end-to-end application performance monitoring. These systems monitor how applications work, gathering data on any issues that arise, such as supporting infrastructure and app dependencies. When anomalies are discovered, rate limiting is activated, and administrators are alerted to the source of the problem with the problematic data.
Security anomalies in web applications: These include any other unusual or suspicious web application activity that might compromise security, such as CSS or DDOS assaults.
Each form of abnormality is detected using continuous, automated monitoring to build a picture of typical network or application activity.
Why Is Detecting Anomalies Important?
Network administrators must be able to recognise and respond to changing operating conditions. Any variations in data security centre or cloud application operating circumstances might indicate excessive levels of business risk. Some divergences, on the other hand, may indicate good growth.
As a result, anomaly detection is critical for extracting critical business insights and ensuring the continuity of vital processes. Consider the following patterns, which all need the capacity to distinguish between normal and deviant behaviour with precision and accuracy:
- An online retailer must forecast whether discounts, events, or new goods will result in sales spikes, putting more strain on its web servers.
- An IT security team must recognise anomalous login patterns and user activities in order to avoid hacking.
- A cloud provider must allocate traffic and services, as well as evaluate infrastructure improvements in light of traffic trends and previous resource failures.
- A well-constructed behavioural model based on evidence may assist users not only describe data behaviour, but also detect outliers and do significant prediction analysis.
Techniques for Detecting Anomalies
When scanning data security for anomalies that are relatively infrequent, the user is bound to come across significant amounts of noise that might be mistaken for aberrant activity. This is because the distinction between aberrant and regular activity is often blurry, and it shifts frequently as malevolent attack patterns refine their tactics.
Anomaly detection algorithms are even more complicated due to the fact that many data patterns are based on time and seasonality. For example, the necessity to break down various trends across time necessitates more complicated algorithms to distinguish between true seasonality and noise or aberrant data.
Conclusion
Various anomaly detection approaches exist for all of these reasons. One may be better than the others for a certain person or data security collection depending on the circumstances. A generative technique builds a model entirely from instances of normal data from a cyber security diploma course, then assesses each test case to see how well it matches the model. A discriminative technique, on the other hand, tries to discriminate between normal and aberrant data groups. In discriminative techniques, both types of data are employed to train algorithms.