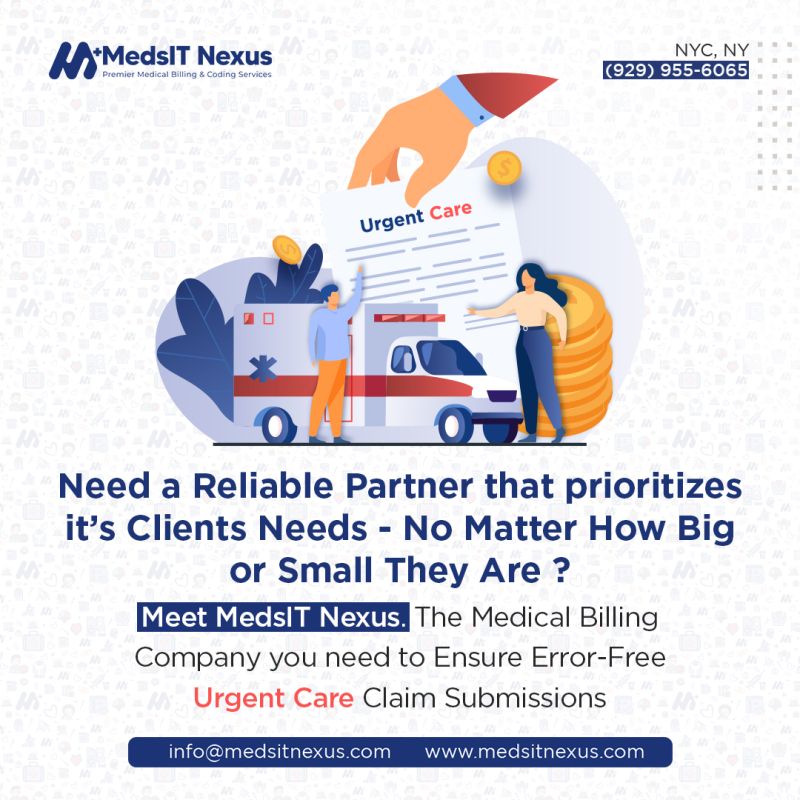
For ODSC West 2021, Sourav Mazumder will be speaking. For more more on trustworthy AI, be sure to view his session, "Operationalization of Models Developed and Deployed in Heterogeneous Platforms." PART 1 of the series may be found here.
Data, data products (such as KPIs, characteristics of machine learning models, insights gleaned from data, etc.), and models can all be used to create AI solutions (e.g. machine learning models, decision optimization models, etc). We will pay particular attention to the governed operationalization of AI models developed using machine learning (ML) methods in the remaining sections of this paper. The other parts of AI solutions, though, might also use the same ideas. For the purpose of simplicity, we will simply refer to ML models for AI solutions as AI models.
Operationalization of AI Models under Control
A paradigm called "governed operationalization of AI models" makes use of people, process, and technology to assist guarantee the reliability of AI solutions utilised in business. The strategy is based on reliable AI ethics and employs data and AI technologies that are integrated with an open and diversified ecosystem. The complete lifecycle of ML Models, from conception to decommission, is covered by governed operationalization of AI models. The procedure and human elements of the same are depicted in the diagram below.
MedsDental is a renowned Dental Billing Company in the united states, equipped of the revenue cycle experts who are highly proficient in delivering fast and the error-free billing services to the dental practices by using the cutting edge technology.
Creation of the Model and Establishment of Candidacy — It is crucial to consider why a reliable AI model is required, what should be utilised to produce it, and how it will be used before beginning to build one. What type of data is going to be used; what algorithm (or type of algorithms) would be most suitable to build the model; what kind of software tool/library/packages should be used; what kind of accuracy, fairness, and drift threshold need to be maintained for the model to be used in production (otherwise re-training would be needed); what kind of accuracy, fairness, and drift threshold need to be maintained for the model to be used in production; and what kind of s/w tool/library/packages
The building of models requires the sourcing of data and the necessary software tools, libraries, and packages. To ensure that no sensitive customer information or internal data was used for the model development, data sourcing needs governance as applicable for the organization/use case (such as data masking, subsetting of datasets, impersonation, and algorithm-specific anonymization). Additionally, it's crucial to comprehend the data's quality, which can be made sure of by data profiling and lineage. To ensure that only reliable (stable, proven, audited) s/w libraries are used to construct the model, packages and libraries used for model creation should also undergo some kind of governance.
Read More: MedsIT Nexus
https://odsc.com/california/#register
Model creation — To construct a model with the proper balance of the model's performance requirements, data scientists often need to test out a variety of methods, features, and hyperparameters. For that, they need to run a number of experiments. From a governance standpoint, it's critical to document each of these trials, their findings, and the justifications for selecting the chosen model. In addition, reusing features from a feature store while creating the models might lead to improved performance management. Therefore, if possible, the use of pre-made (and used) features can also be required during the model development process.
Independent Model Validation — Just as with traditional software, AI models require model validation by an independent team that is separate from the team that created the model. Through thoughtful test design, the independent validation team should provide a unique validation dataset (blind datasets). They ought to be able to evaluate the model's performance in a number of areas, including fairness, robustness (using drift), interpretability, throughput, and others. The threshold of the relevant metrics determined during the model inception phase should be used to compare these results with. Unless the test results fall within the acceptable range of the thresholds, the model owner should not give the model permission to be used in production.Managing the billing process accurately is not easy as providers might face hurdles in revenue cycle management. Moreover, Net Collection Rate below 95% shows that your practice is facing troubles in the billing process. To eliminate all these hurdles and maintain your NCR up to 96%, MedsIT Nexus Medical Coding Services are around the corner for you so that your practice does not have to face a loss.
Production Model Deployment – Validated models are implemented in production. Now, a variety of applications can make score queries to the model over technology-neutral standard protocols like Rest/HTTP to obtain predictions. There are two possible deployment types: batch and online (synchronous access) (asynchronous access). To access a model synchronously for a single prediction request, a model execution runtime must be running continuously throughout online deployment (or a small set of prediction requests, aka micro-batch). This is frequently employed in use situations where the model must make predictions in real-time, such as the detection of chatbot intent and the prediction of online transaction fraud. An infrastructure that can spawn the runtime on demand and suspend it once predictions for all batch scoring requests have been created is necessary for batch deployment of models. With use cases that may wait for a condition before the predictions are made, this mode is generally utilised to obtain predictions for a high volume of score requests. For instance, the daily determination of clients with a high propensity to leave, risk factor forecasts for loans requested every day, etc. In both situations, it's critical to keep an eye on who has access to the model execution environment for governance reasons. It is essential to monitor (or prevent) unwanted access to the environment, execution faults, response throughput and latency, and model performance (accuracy, drift, fairness, etc.). Before making it available to business applications and clients, the model's execution environment needs to be built to accommodate all of these monitoring requirements. Additionally, the security of model binaries, scoring data security, and the model serving environment (none should be allowed to spawn or remove), all need to be addressed.