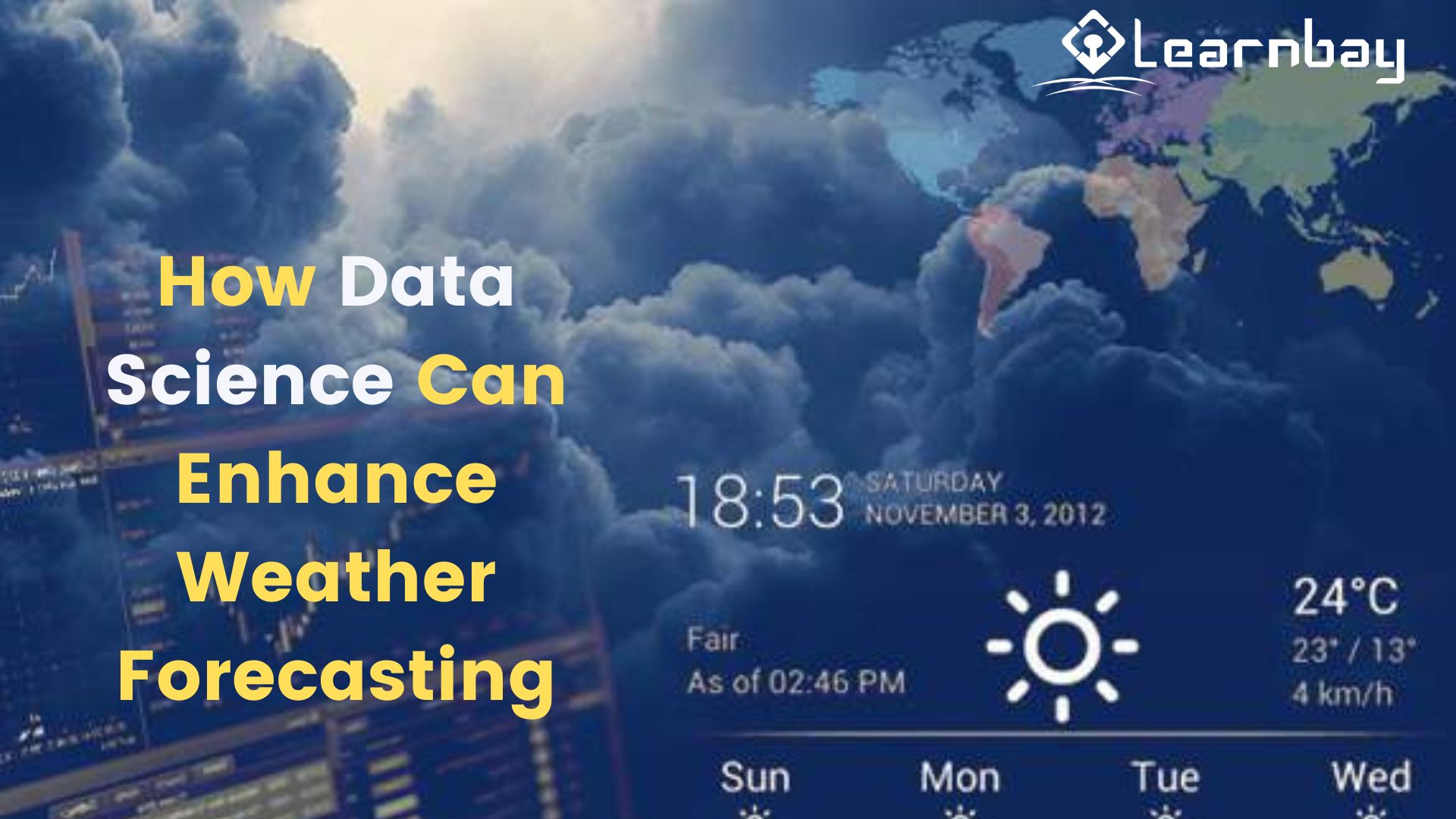
Weather forecasts are far from perfect, as anybody who has carried an umbrella under the brightest of skies knows. They cannot provide 100% accuracy on climatic conditions and are notorious for being inaccurate even in the near term. Nonetheless, projections have gotten increasingly exact in recent years, even though most people regard them as a possibility rather than an educated prediction.
There's a compelling reason for this: -
The use of data science to improve existing weather forecasting models. True, these models have always been based on data collection and analysis. However, technical improvements in data science introduced by Python, R, and Java development organizations can usher in a new age in weather forecasting. Let's go through it in greater depth, but first, let's talk about weather forecasting.
How modern Weather Forecasting works
Though we all like to point fingers at the weather forecasters who share their predictions on the news, the process of predicting the weather is incredibly hard. This procedure is built on two major components. On the one hand, a massive volume of data is arriving from several sources. On the other hand, weather models and analysis attempt to make sense of all the incoming data.
Several instruments and technologies collect weather information. There's a lot of data floating about, from simple devices like thermometers, barometers, and anemometers to complex equipment like weather balloons and radar systems to environmental and deep-space satellites. To know more about data science tools and techniques utilized in weather prediction, visit the data science course in Chennai led by industry experts.
When it comes to predictions, it's very obvious that data availability isn't an issue. As a result, we must investigate the weather models utilized to find patterns and insights from such data sets. Given that they offer the greatest results while operating in real-time and the environment continually changes, long-term estimates are difficult to come by.
In this setting, where a large amount of data must be continuously analyzed for improved predictions, it's impressive that these models can provide seven-day weather forecasts with an estimated 80% dependability. Is it conceivable, though, to bring it closer to 100%? That is the issue that Python, R, and Java developers attempt to solve through novel technological techniques.
The future of weather forecasting
If data science is currently a well-established process in weather forecasting, it is up to innovative ways in it to increase that by 80%. And no ally is more powerful than artificial intelligence (AI) and one of its most potent subdivisions, machine learning (ML). Meteorological models can benefit substantially from implementing ML-based algorithms since this technology can handle massive volumes of weather data and adapt itself for more accurate forecasts as it is employed.
The benefit of utilizing machine learning for weather forecasting is that it can make instant comparisons and discover trends. Python, R, and Java development services are creating new platforms and solutions that can compare data from weather stations, radars, and satellites to previous weather reports. As a result, the program is taught to filter out mistakes and inconsistencies based on previously known data and present situations.
There's more to it. Weather forecasting might become increasingly more precise over time if it moves beyond machine learning and into deep learning. This is because deep learning algorithms process and analyze data as the human brain does. The only (significant) difference is that these algorithms work considerably quicker than humans ever could.
There are already instances demonstrating the possibilities that data science combined with the machine and deep learning may bring to the table. For example, IBM purchased The Weather Company and utilized its data to feed Watson, their famed AI engine. Deep Thunder, along with very accurate hyper-localized predictions, was formed as a result.
Another example is Bayer's Climate Corporation, which extensively invests in AI for agricultural weather forecasting. Their Fieldview system, which uses machine learning algorithms, gives weather projections that considerably aid farmers' decision-making processes.
Smartphones are the final technology that has found its way into weather forecasting. Yes, mobile devices are helping to advance data science. How so? Weather services can tailor-made predictions with high degrees of accuracy by gathering data from their users' unique locations. Users who use weather applications are therefore linked into a feedback loop that supplies them with accurate weather information, which improves as a result of their usage of the app in the first place.
What next
Weather forecasting has seen significant changes in the previous several decades. However, there is more in store for the sector, especially with the addition of AI and ML. The objective is to improve prediction accuracy. Thus there is more work to be done.
The growth of hyper-localized forecasting and nowcasting is expected to continue in the future years. This will be due to the increased usage of machine learning models and deep learning solutions and the ever-increasing use of smartphones. This will be integrated to provide more precise data to feed increasingly complex systems.
We'll likely never be able to make forecasts with 100% accuracy. However, even a 1% improvement in existing projections is a significant step forward, not just for everyday weather prediction but also for forecasting severe climate calamities. In this regard, data science appears to be the ideal ally for improving existing projections and providing improved estimates for everyone. Are you a data scientist aspirant? Sign up for data science in Chennai and become a certified data science professional.