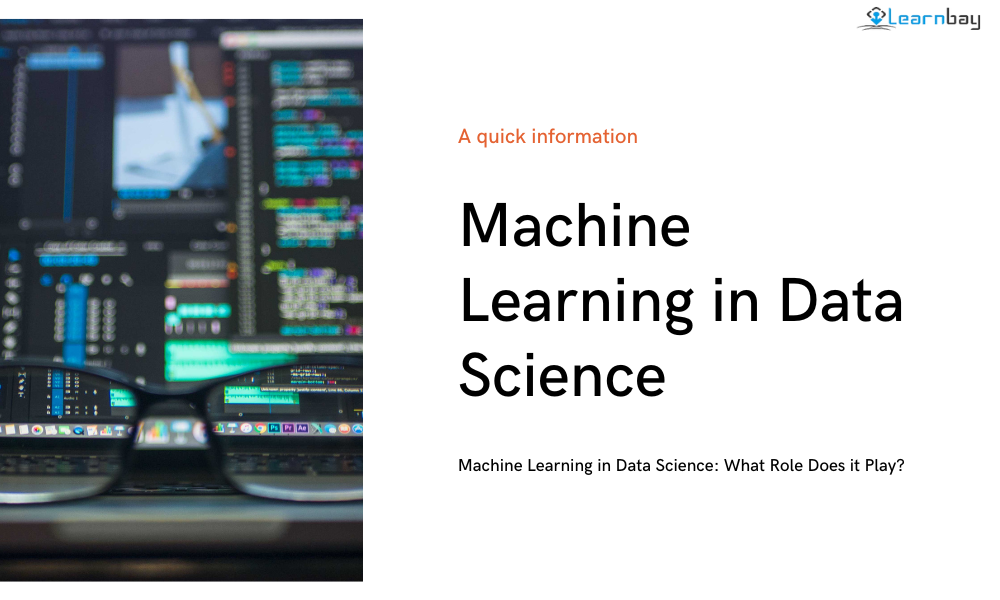
The term "machine learning" is currently quite popular. It's interesting to realize that machine learning has existed for a while without your knowledge. Have you ever wondered how YouTube chooses which video to show you next?
It examines the videos you're watching, the channel from which they came, their length, and the subjects they cover. So, before proposing the next video to you on YouTube, all these criteria are considered. In other words, YouTube "learns" from your viewing patterns and recommends similar videos. You have been exposed to instances of machine learning for many years. Check out the online Data Science course in Bangalore, if you are curious to learn about this exciting field.
Machine Learning is one of a large range of topics covered by data science, as you are aware. In order to analyze data and derive relevant insights, data scientists use a variety of areas and techniques, including statistics and artificial intelligence.
How Does Machine Learning work?
Machines can now be trained using a data-driven method. If you consider artificial intelligence the main umbrella, then machine learning is a subset of artificial intelligence on a larger scale. The capacity for machines or computers to autonomously learn from data using a set of algorithms is known as machine learning.
Machine learning is based on the principle that you can educate and train machines by giving them data and specifying characteristics. When given fresh, pertinent data, computers learn, grow, adapt, and evolve independently without the need for explicit programming. Machine learning is a relatively limited field without data.
The Machine examines the dataset, spots patterns in it, automatically picks up on patterns from behavior and predicts outcomes.
Internet recommendation engines, whether for YouTube video recommendations or Facebook friend recommendations, employ machine learning technology to provide the user with recommendations that are pertinent to their needs.
Google's Autonomous Driving Vehicle, one of the most current technologies, also uses machine learning algorithms to comprehend patterns and definitions, learn automatically, and operate.
What are the Data Science Applications of Machine Learning?
Real-Time Navigation
Google Maps is one of the most widely used Real-Time Navigation systems. But have you ever questioned why you are taking the fastest route despite being in normal traffic? The database of Historical Traffic Statistics and the information gathered from users of this service at the time are to blame. Every person who makes use of this service helps to improve the accuracy of this program.
As you launch the application, it continuously transmits data to Google, providing details on the route taken and the flow of traffic at any given time of day. Because so many people use the app often, Google has amassed a sizable database of traffic information that it can use to watch traffic in real-time and make predictions about what will happen if you stick along the same path.
Image Recognition
One of the most popular uses of machine learning in data science is image recognition. Image recognition is utilized to identify things like people, locations, and objects. The most common applications for this software are Facebook's automatic friend tagging suggestions and face recognition on smartphones.
Product Recommendation
eCommerce and entertainment firms like Amazon, Netflix, Hotstar, etc., heavily rely on product recommendations. To provide suggestions for products and services you might be interested in; they use a variety of Machine Learning algorithms on the information they have about you. Refer to the Machine learning course in Bangalore, to gain profound knowledge of popular ML algorithms used by data scientists.
Speech Recognition
Voice recognition is the process of turning spoken words into written ones. This material can be described using words, syllables, sub-word units, or characters. Siri, Google Assistant, YouTube Closed Captioning, etc., are a few popular examples.
Challenges of Machine Learning in Data Science
The face of industries has been completely transformed by machine learning in data science. It has aided businesses in making wise decisions that would help them expand. Yet, it still confronts a few difficulties that a data scientist must consider. The Top 3 Challenges of Machine Learning in Data Science are listed below:
Lack of Training Data
The foundation of any machine learning model is data. Nonetheless, obtaining tagged data is quite expensive and challenging. Every data scientist struggles with the problem of training a machine learning model without a lot of data. Transfer Learning is one approach to addressing this issue. It allows the model to use information from previously learned tasks and apply it to new, related tasks. Another approach to resolving this issue is self-supervised learning. It presents a fantastic potential for better using vast volumes of unlabeled data.
Discrepancies between Data
The second issue is that there are frequently some inconsistencies between the training and production sets of data. Occasionally a model will perform well in a prototype environment but fall short in real-world situations.
For instance, the model could perform well in one nation but poorly in another owing to regional variations, perform well during the winter but poorly during the summer due to seasonal variances, perform well on mobile devices but poorly on desktop computers due to user preferences, etc. You must be extremely attentive while gathering your training data in order to fix this issue. You must often update your model to keep it as near to your target domain as feasible.
Model Scalability
One of the greatest difficulties that industries confront is this. As a data scientist, it is your responsibility to ensure that your model is quick and compact. Post-Training Quantization is one approach to this issue. With a slight loss in model fidelity, it is a conversion technique that shrinks the model's size while also increasing CPU and hardware accelerator latency.
5 Major Steps of Machine Learning in Data Science Life Cycle
Step 1 - Data Collection
It is regarded as the first stage in machine learning. It is crucial to get accurate and pertinent data since the quantity and quality of the data directly affect how well your machine-learning model performs. This dataset is further utilized for training your data model, as was covered in the previous section.
Step 2 - Data Preparation
The initial stage in the total data preparation process is data cleaning. This is a crucial step in getting the data suitable for analysis. Data preparation ensures that there are no incorrect or inaccurate data points in the dataset. Also, the data must be standardized into a single format. Furthermore, divided into two sections, the dataset may be utilized to train your data model and assess the effectiveness of the trained model.
Step 3 - Training the Model
The process of "learning" begins here. The output value is predicted using the Training dataset. This output is bound to deviate from the required value at the initial iteration. Yet a "Machine" becomes better with use. After making certain tweaks to the initialization, the procedure is done again. The training data is utilized to raise your model's prediction precision gradually.
Step 4 - Model Evaluation
It's time to assess your model's performance when you've finished training it. The dataset set aside during the data preparation step is used in the assessment process. The model has never been trained with this data. As a result, testing your data model against a fresh dataset will help you predict how it will perform in practical situations.
Step 5 - Prediction
The fact that your model has been trained and tested does not imply that it is flawless and prepared for deployment. The settings can be adjusted to enhance the model further. The ultimate stage of machine learning is prediction. Your data model is implemented at this stage, and the computer uses what it has learned to respond to your inquiries.
Conclusion
Organizations place a lot of emphasis on leveraging data to enhance their offerings. Data science is nothing more than data analysis without machine learning. Generally, data science and machine learning co-exist together. Machine learning simplifies the tasks for data scientists by automating them. Machine learning will soon play a significant role in analyzing a vast quantity of data. Further, knowing Machine Learning (ML) will increase the efficiency of Data Scientists. If you are passionate about becoming a data scientist, Learnbay has exclusive courses in store for you. Register in the best data science course in Pune, master the skills, work on 15+ real-world projects, and become a certified data scientist now!