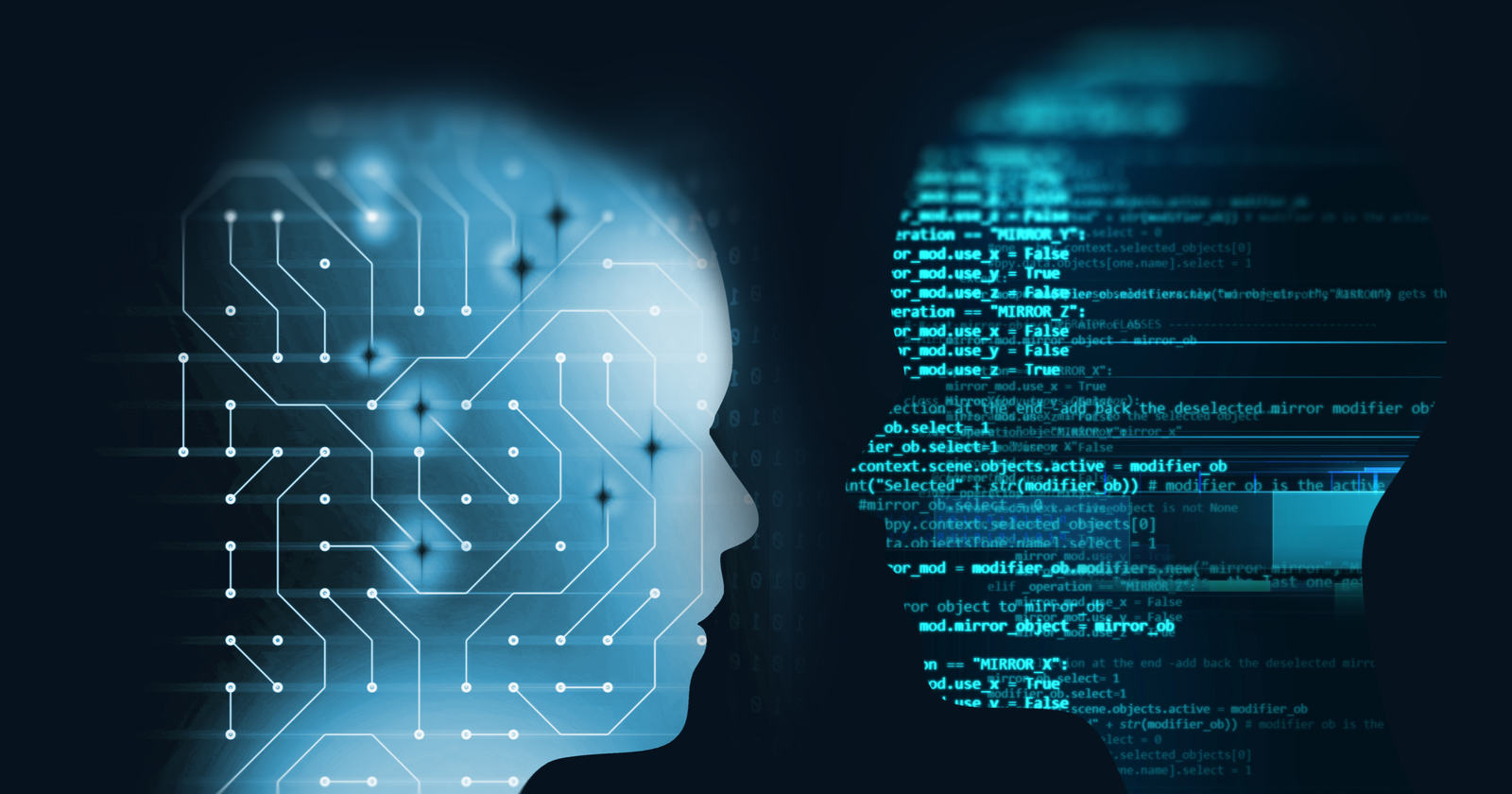
Chebyshev's inequality is a fundamental theorem in probability theory and statistics that provides a bound on the probability that a random variable deviates from its mean by a certain amount. This inequality is named after the Russian mathematician Pafnuty Chebyshev, who first introduced it in 1867.
Chebyshev's inequality is particularly useful in data science because it allows us to make statements about the spread of a probability distribution without assuming anything about its shape or parameters. This makes it a powerful tool for understanding the behavior of random variables in a wide range of settings.
Several reputed institutes now offer the data science online course online too.
STATEMENT OF CHEBYSHEV'S INEQUALITY
Chebyshev's inequality states that for any random variable X with finite mean μ and finite variance σ^2, the probability that X deviates from its mean by more than k standard deviations is at most 1/k^2:
P(|X-μ|≥kσ)≤1/k^2
where k is any positive real number greater than 1.
In other words, the probability that X deviates from its mean by more than k standard deviations is bounded by 1/k^2. This bound holds for any probability distribution, regardless of its shape or parameters.
INTUITION BEHIND CHEBYSHEV'S INEQUALITY
The intuition behind Chebyshev's inequality is that the variance of a random variable measures how much it deviates from its mean. The larger the variance, the more spread out the probability distribution is, and the more likely it is that the random variable will deviate from its mean by a large amount.
Chebyshev's inequality provides a way to quantify this intuition. The inequality states that the probability of a large deviation from the mean is inversely proportional to the square of the number of standard deviations. In other words, the probability of a large deviation decreases rapidly as we move away from the mean.
Applications Of Chebyshev's Inequality
Chebyshev's inequality has a wide range of applications in data science and machine learning, including:
Outlier detection: Chebyshev's inequality can be used to detect outliers in a dataset. If a data point deviates from the mean by more than a certain number of standard deviations, it is considered an outlier. Chebyshev's inequality provides a way to set a threshold for what constitutes an outlier.
Confidence intervals: Chebyshev's inequality can be used to construct confidence intervals for a sample mean. The inequality provides a bound on the probability that the sample mean deviates from the true population mean by more than a certain amount.
Data cleaning: Chebyshev's inequality can be used to clean data by identifying values that are unlikely to be valid. For example, if a data point deviates from the mean by more than 3 standard deviations, it may be a data entry error or a measurement artifact.
Quality control: Chebyshev's inequality can be used in quality control to ensure that a manufacturing process is producing products within certain specifications. The inequality can be used to set tolerances for how much a product can deviate from its target value.
The data science course fees may go up to INR 6 lakhs.
LIMITATIONS OF CHEBYSHEV'S INEQUALITY
One of the main limitations of Chebyshev's inequality is that the bound it provides is often lose. The inequality states that the probability of a deviation from the mean by more than k standard deviations is at most 1/k^2. However, in practice, the probability of a large deviation is often much smaller than this bound. For example, for a normal distribution, the probability of a deviation from the mean by more than 3 standard deviations is less than 0.003, while Chebyshev's inequality gives a bound of 1/9 or approximately 0.11. This means that Chebyshev's inequality may overestimate the probability of a large deviation, and caution should be exercised when interpreting the bound it provides.
Another limitation of Chebyshev's inequality is that it does not tell us how likely a deviation is. The inequality provides a bound on the probability of a deviation, but it does not tell us whether the deviation is likely or unlikely. For example, if the probability of a deviation from the mean by more than 3 standard deviations is 0.01, this may still be considered a significant deviation if the consequences of such a deviation are severe. Conversely, if the probability of a deviation is very small, but the consequences of such a deviation are minor, it may not be worth taking extra precautions to prevent it.
A related limitation of Chebyshev's inequality is that it assumes nothing about the shape or parameters of the probability distribution. While this is often a strength of the inequality, it can also be a weakness in some cases. For example, if we know that a random variable follows a normal distribution, we can use this information to derive a tighter bound on the probability of a deviation than Chebyshev's inequality provides. In such cases, it may be more appropriate to use a distribution-specific bound rather than relying on Chebyshev's inequality.
Finally, it should be noted that Chebyshev's inequality is a one-sided bound. That is, it only provides a bound on the probability of a deviation in one direction (either above or below the mean). In some cases, we may be interested in a two-sided bound, which gives a bound on the probability of a deviation in either direction. In such cases, other methods may be more appropriate, such as the Hoeffding's inequality or the Bernstein's inequality.
Despite these limitations, Chebyshev's inequality remains a valuable tool in data science and statistics, providing a simple and general way to bound the probability of a deviation from the mean. However, it is important to keep in mind the limitations of the inequality and to use it appropriately in each situation. In particular, caution should be exercised in future too when interpreting the bound it provides, as it may be looser than the actual probability of a deviation.
Several reputed institutes offer the data science course in India.