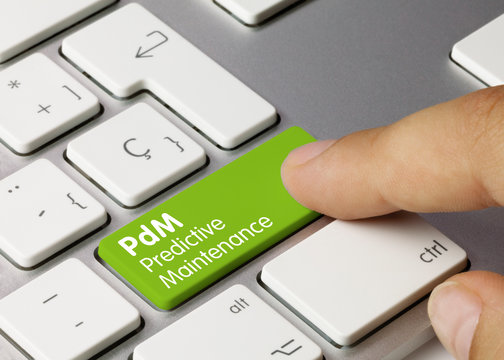
Data-Driven Predictive Maintenance
The manufacturing industry has shown considerable interest in data-driven predictive maintenance. This technique is a step ahead of the traditional reactive and preventive maintenance models. The data-driven approach uses machine data to predict equipment failures, instead of relying on pre-determined maintenance plans.
The data-driven predictive maintenance technique is based on algorithms that use machine learning techniques to analyze massive amounts of data generated by equipment. Through this analysis, the algorithms can detect potential problems, plot trends, and suggest the best course of action. This proactive approach helps manufacturing operations reduce equipment downtime and improve overall quality.
One significant advantage of data-driven predictive maintenance is its ability to detect anomalies in operational data that predict equipment failure. The data-driven predictive maintenance system establishes a baseline for operational data and continuously monitors data within predefined parameters. If the data moves beyond these parameters, predictive maintenance can identify potential system failures.
Data-driven predictive maintenance is not a one-time investment. It is a continuing process that requires the collection of performance and fault data from equipment and the use of the data to refine the algorithms continually. Predictive maintenance programs are essential in today's manufacturing industry, and it is essential to rely on the best technologies to ensure the highest levels of uptime and equipment availability.
Machine Learning in Predictive Maintenance
Machine learning (ML) lies at the heart of predictive maintenance. It describes the complex algorithms used by predictive maintenance software to identify equipment issues before they occur. In a manufacturing environment, machine learning algorithms can analyze data streams from operations and equipment to make inferences about possible equipment failure outcomes.
The key advantage of using ML in predictive maintenance is that it can identify patterns in the data, creating a model that can act as a predictive predictor of asset failure. This model can then be used to guide maintenance schedules and decisions, thereby improving performance and avoiding unnecessary downtime.
The predictive analytics software used in data-driven predictive maintenance usually utilizes unsupervised ML techniques to learn from equipment data, including time-series data such as temperature, pressure, and energy consumption, that are not previously labeled nor classified. The unsupervised ML techniques can detect trends and anomalies in the equipment data and can identify potential problems and their root causes, without the need for manual adjustments.
The algorithms used for machine learning in predictive maintenance are not limited to traditional statistical models such as linear regression and decision trees. Machine learning techniques such as deep learning and reinforcement learning are also used in the analysis of complex systems.
A combination of supervised and unsupervised machine learning techniques can reduce the amount of time it takes to train predictive maintenance models. These algorithms can learn from previous data sets even as they continue to collect real-time data from the equipment within a manufacturing environment.
Future Trends in Predictive Maintenance
Here are some of the significant trends that we expect to influence the adoption of predictive maintenance for the manufacturing industry in the coming years.
1. Internet of Things (IoT)
IoT has played a significant role and will continue to do so in predictive maintenance. The technology provides real-time data from sensors placed in various equipment, allowing maintenance professionals to keep a hawk's eye on the workings of their devices. IoT sensors can detect faults before they even occur.
IoT technology has the potential to improve the efficiency of predictive maintenance algorithms given its ability to generate more data than ever before. Manufacturers can use the data generated by IoT sensors to make more informed decisions in real-time about the maintenance practices, equipment optimization, and feedback to equipment suppliers for further improvements.
2. Cloud Computing
Cloud computing has enabled manufacturers to automate data replication, collect data from various sources in real-time, and store it securely. Cloud computing means manufacturers can employ predictive maintenance models without needing to spend on capital-intensive infrastructure, primarily through the use of cloud-based analytics services.
Cloud computing provides an opportunity for manufacturers to scale their predictive maintenance offerings easily and inexpensively. This ability to scale on a global scale is especially appealing to manufacturers with worldwide operations.
3. Edge Computing
Edge computing allows companies to run applications and procedures directly on the device without accessing the cloud or a centralized server. This process means that manufacturers can use predictive maintenance models directly on their equipment without relying on cloud technology.
Edge computing reduces latency issues by processing data at the equipment level and can be used in complex manufacturing environments, especially industrial sites where distributed sensors are used to gather data from a large number of machines.
4. Artificial Intelligence (AI)
AI has the potential to transform the predictive maintenance process completely. AI systems can analyze vast amounts of data in real-time without human intervention, enabling them to identify patterns and develop predictive maintenance models far beyond what is possible with conventional statistical techniques.
AI-based predictive maintenance models have the potential to integrate with other artificial intelligence systems such as robotics and automated control systems to create a fully integrated and intelligent industrial ecosystem.
Conclusion
In conclusion, predictive maintenance has become a critical component of modern manufacturing operations. With the help of data-driven approaches and machine learning techniques, manufacturers can analyze vast amounts of data from their equipment to spot and correct problems before they affect their business.
Predictive maintenance's future holds exciting opportunities with new technologies such as IoT, cloud computing, edge computing, and AI systems. These technologies will enable manufacturers to continue developing, improving, and optimizing predictive maintenance models, helping to ensure that manufacturing operations remain efficient, effective, and profitable.