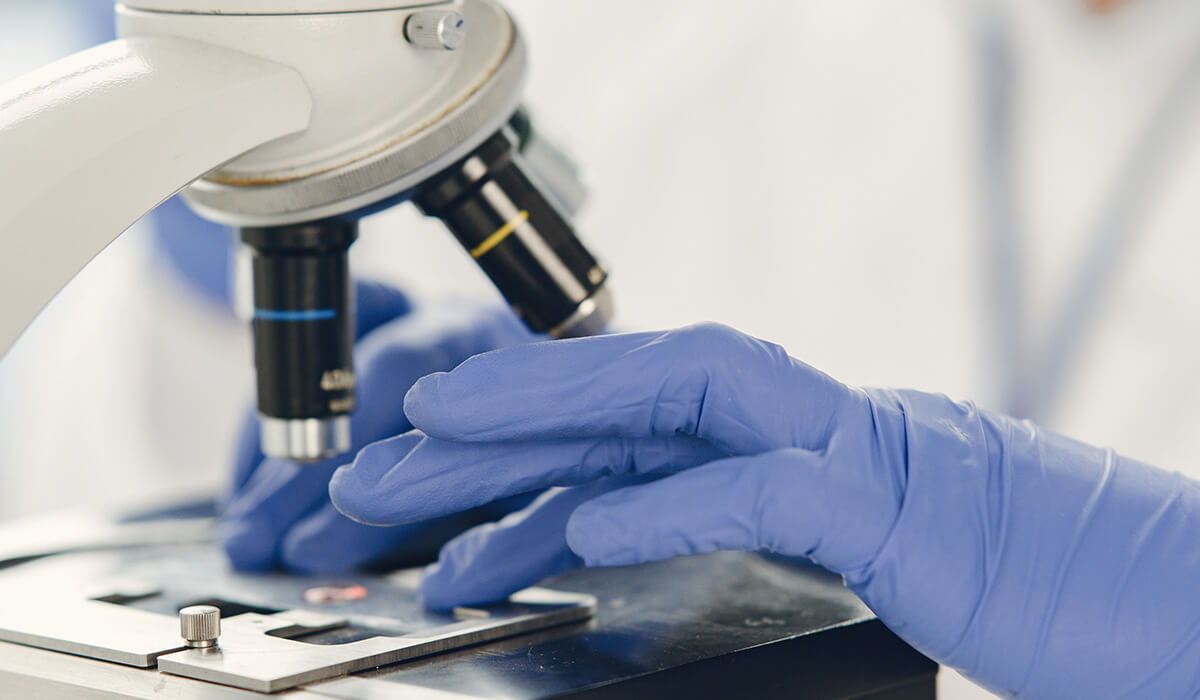
Introduction:
In the realm of drug discovery and development, in vitro screening plays a vital role in identifying potential drug candidates for further evaluation. However, the traditional methods of in vitro screening have limitations, including time-consuming processes, high costs, and challenges in accurately predicting the efficacy and safety of drug candidates. To overcome these hurdles, scientists and researchers have turned to computational approaches, leveraging the power of artificial intelligence, machine learning, and data analytics, to enhance the efficiency and accuracy of in vitro screening.
In this blog post, we will explore how computational approaches are integrated with In vitro screening, focusing on the emerging field of in vitro ADME screening, and delve into the significant advancements they offer to the drug discovery process.
I. The Basics of In Vitro Screening:
Before diving into the integration of computational approaches, it is important to understand the fundamentals of in vitro screening. In vitro screening involves conducting experiments on isolated cells, tissues, or biochemical components outside of a living organism to study the effects of various compounds, such as potential drug candidates. It serves as a preliminary step in identifying lead compounds with desired properties before progressing to more complex in vivo studies. However, the traditional in vitro screening methods have limitations in terms of throughput, accuracy, and predictability. This necessitates the integration of computational approaches to overcome these challenges effectively.
II. Computational Approaches in In Vitro Screening:
a) Virtual Screening: Computational models can be utilized to virtually screen vast databases of chemical compounds and identify potential drug candidates with desired properties. By applying molecular docking, machine learning algorithms, and structure-based drug design techniques, virtual screening significantly accelerates the initial phase of the drug discovery process.
b) Quantitative Structure-Activity Relationship (QSAR) Modeling: QSAR models use statistical algorithms to establish relationships between the chemical structures of compounds and their biological activities. By analyzing the chemical properties and structures of known active compounds, QSAR models can predict the activity of untested compounds, aiding in the selection of promising candidates for further in vitro screening experiments.
c) High-Throughput Screening (HTS): High-throughput screening involves the rapid testing of large compound libraries using automated robotic systems. Computational approaches assist in the design of efficient screening protocols, analysis of the obtained data, and the identification of hit compounds for further investigation. This integration improves the efficiency of the screening process by reducing costs, time, and resource requirements.
III. The Emergence of In Vitro ADME Screening:
ADME (Absorption, Distribution, Metabolism, and Excretion) studies are crucial in drug development to evaluate the pharmacokinetic properties and safety profiles of potential candidates. In vitro ADME screening involves assessing how a compound is absorbed, distributed, metabolized, and eliminated within an organism. Computational approaches play a pivotal role in streamlining and enhancing in vitro ADME screening.
a) Predictive ADME Models: Computational models trained on large datasets of known ADME properties can accurately predict the absorption, distribution, metabolism, and excretion profiles of new compounds. These models assist in selecting drug candidates with optimal ADME characteristics, ultimately improving the chances of success in later stages of drug development.
b) Pharmacokinetic Modeling: Pharmacokinetic models integrate data from in vitro ADME screening experiments with other pharmacological and physiological information to predict drug concentrations in different tissues over time. Such models help researchers understand the kinetics of drug absorption, distribution, metabolism, and excretion, aiding in dose optimization and reducing the risk of adverse effects.
Conclusion:
In vitro screening serves as a critical phase in the drug discovery process, but its limitations necessitate the integration of computational approaches to enhance efficiency and accuracy. By leveraging virtual screening, QSAR modeling, high-throughput screening, and the emerging field of In vitro ADME screening, researchers can make significant advancements in identifying promising drug candidates. Computational approaches offer faster and more cost-effective methods for screening large compound libraries, predicting compound activity, and evaluating ADME properties. As technology continues to evolve, these approaches will become even more indispensable, accelerating the drug discovery process and leading to the development of safer and more effective medications.
Incorporating computational approaches in in vitro screening and in vitro ADME screening presents a paradigm shift in the field of drug discovery, revolutionizing the way potential drug candidates are identified and evaluated. By harnessing the power of computational methods, scientists can navigate through the vast chemical space more efficiently and accurately, ultimately leading to the discovery of novel therapies that have the potential to transform healthcare.