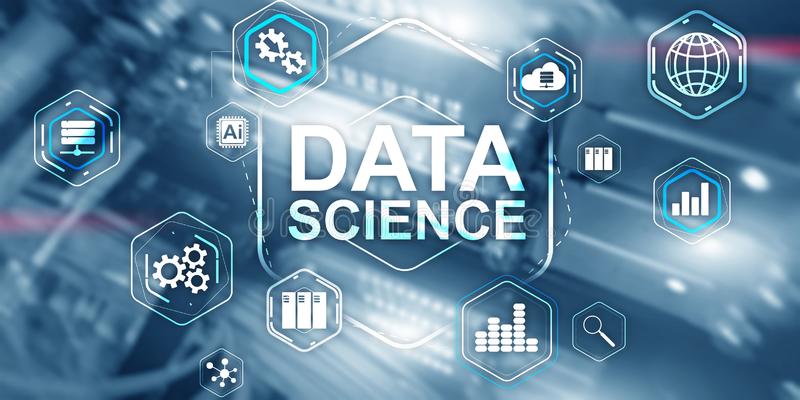
To analyse and make sense of data, models are necessary in the fields of machine learning and statistics. When it comes to representing and learning from data, parametric and non-parametric models take quite different methods. Parametric and non-parametric models are contrasted in detail in this article. Readers will be able to pick the most suitable modelling technique for various kinds of data and problem domains after becoming familiar with their features, strengths, and limitations.
A data science online course can be helpful to get a better understanding of this subject.
Modelling using Parameters
Strong assumptions regarding the data distribution are required for parametric models. They are characterised by a small number of parameters that are always the same, no matter how big the dataset becomes. Linear regression, logistic regression, and Gaussian Naive Bayes are all types of parametric models. Distribution specification and parameter estimation are prerequisites for these models. Parameter estimation completes the model definition, allowing for uncomplicated prediction.
Statistics Without Parameters
When compared to parametric models, non-parametric models assume less about the nature of the data's distribution. They are adaptable and can learn from new data structures and connections. The size of a dataset does not limit the number of parameters in a non-parametric model. Decision trees, random forests, support vector machines (SVM), and k-nearest neighbours (KNN) are all types of non-parametric models. Intricate data structures may be captured by these models without the need to provide a functional form.
Complexity and the Ability to Change
The construction of parametric models is more straightforward, and they often rely on a set of predetermined assumptions about the nature of the data. The model's adaptability is reduced, although estimate and interpretation are facilitated by these assumptions. However, non-parametric models are more adaptable and may better capture subtle interrelationships in the data and algorithms. They are well-suited to domains with complicated and nonlinear interactions because they can learn sophisticated patterns without making strong assumptions.
Instruction and Application
The parameters of a parametric model must be estimated using the training data. Optimization methods are used in this estimating procedure in order to minimize a target objective function. The model's predictive ability greatly improves when the parameters have been calculated. In contrast, non-parametric models may be trained with little data and then automatically adjust their level of complexity to match what was seen in the data. When the underlying distribution is uncertain or complicated, this flexibility may improve generalization performance.
Exchange of Bias for Variability
An essential idea in modelling is the trade-off between bias and variation. Due to the assumptions made about the data distribution, parametric models may have low variance but significant bias. The model's capacity to pick up nuanced connections may suffer as a result of this bias. Due to their adaptability, non-parametric models often have low bias but are more prone to large variation, particularly when working with insufficient training data. For the best modelling performance, it is essential to strike a balance between bias and variance.
Need for Data and Capacity to Grow
Parameter estimation in parametric models often requires less data. Because they make an assumption about the distribution, they may estimate the parameters with little data. On the other hand, non-parametric models need more information before they can understand complicated associations. Due to their flexible character, non-parametric models scale effectively with increasing dataset sizes. This adaptability, nevertheless, may take more time and space in the computer's memory.
Capacity for Understanding and Explanation
Results from parametric models are often interpretable since parameters have well-defined roles and are straightforward to comprehend. This interpretability is especially useful in fields where clarity and explanation are crucial, such as healthcare and finance. Due to their complicated nature, non-parametric models are not always easily interpretable, which may make it hard to fathom the reasoning behind a given conclusion. Non-parametric models' behaviour may be understood, however, with the help of methods like feature significance measures and local explanations.
Conclusion
Different strategies for modelling and inferring from data may be found in both parametric and non-parametric models. The strong assumptions about the data distribution that parametric models make allow for straightforwardness, interpretability, and efficient estimation. Although non-parametric models are more computationally intensive and may need bigger data sets, they provide more flexibility and adaptation to complicated data patterns. Practitioners may choose the most suitable modelling strategy by considering the features of the dataset, the modelling aims, and the need for interpretability.
The data science course fees can go up to INR 3 lakhs.