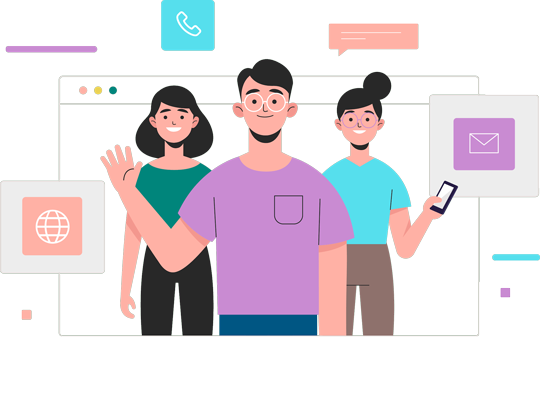
In an age dominated by data-driven decision-making, businesses are constantly seeking ways to leverage technology to gain a competitive edge. Artificial Intelligence (AI) and synthetic data are two powerful tools that have emerged at the forefront of this transformation. The fusion of AI and synthetic data holds the promise of enhancing business analysis in unprecedented ways, but it also comes with its own set of limitations and challenges. In this article, we will explore the benefits and limitations of AI and synthetic data in the realm of business analysis.
The Power of AI in Business Analysis
Artificial Intelligence, often regarded as the "brain" of modern analytics, has revolutionized the way businesses analyze data. Here are some of the key benefits:
Automation and Efficiency:
AI-driven tools, including machine learning algorithms and natural language processing, emphasized in business analytics training in Hyderabad, can quickly analyze vast datasets, saving businesses time and resources. Tasks that used to take weeks can now be completed in a matter of hours, allowing for more in-depth and timely analysis.
Pattern Recognition:
AI, as taught in a business analytics course in Pune, excels at uncovering hidden patterns and trends within data that might go unnoticed by human analysts. This is especially valuable in identifying market opportunities, predicting customer behavior, and optimizing supply chain operations.
Personalization:
AI can tailor business analysis to specific customer segments, offering personalized recommendations and insights. This level of personalization can improve customer experiences and drive revenue growth.
Predictive Analytics:
AI models, as recognized in business analytics certification, can make accurate predictions about future trends, helping businesses make informed decisions. For example, in the finance industry, AI is used to predict stock market movements, while in healthcare, it aids in diagnosing diseases at an early stage.
Real-time Insights:
AI can process data in real-time, enabling businesses to react swiftly to changing market conditions. This agility is critical for industries such as e-commerce, where trends evolve rapidly.
The Role of Synthetic Data in Business Analysis
While AI is a powerful tool, it is only as good as the data it is trained on. This is where synthetic data comes into play. Synthetic data is artificially generated data that mimics the characteristics of real data. Here's how synthetic data complements AI in business analysis:
Data Privacy and Security:
One of the most significant advantages of synthetic data, as highlighted in teachings at a prestigious business analytics institute, is its ability to protect sensitive information. Businesses can use synthetic data for AI model training and analysis without exposing real customer data, ensuring compliance with data privacy regulations like GDPR.
Data Augmentation:
Synthetic data, as covered in a comprehensive business analytics training course hyderabad, can supplement existing datasets, addressing issues related to data scarcity. It can help create more robust and diverse training datasets for AI models, improving their accuracy and generalization.
Scenario Testing:
Businesses can use synthetic data to create various scenarios for testing and validating their AI models. This allows for better model evaluation and fine-tuning before deployment.
Competitive Advantage:
Using synthetic data can give businesses a competitive advantage by enabling them to experiment with new data sources, test hypotheses, and innovate without relying solely on costly, real data acquisition.
Cost Savings:
Acquiring and maintaining real data can be expensive. Synthetic data offers a cost-effective alternative for businesses to train and test AI models.
The Limitations and Challenges of AI and Synthetic Data
While the benefits are substantial, it's crucial to recognize the limitations and challenges associated with AI and synthetic data:
Data Quality and Bias:
AI models, as taught at a reputable business analytics training institute in pune, can perpetuate biases present in training data, which can have ethical and legal implications. Synthetic data generation also relies on real data, potentially inheriting the same biases. Careful data curation and bias mitigation strategies are essential.
Expertise and Resources:
Implementing AI and synthetic data solutions requires a skilled workforce and investment in technology infrastructure. Not all businesses have the necessary resources or expertise to leverage these tools effectively.
Data Security:
While synthetic data offers enhanced data privacy, it is not immune to cyber threats. Businesses must still ensure robust security measures to protect their synthetic data assets.
Accuracy and Realism:
Synthetic data, while useful, may not always capture the full complexity and nuance of real-world data. The challenge is to ensure that synthetic data remains realistic and representative of the domain it aims to emulate.
Evolving Regulations:
Data privacy regulations are constantly evolving. Staying compliant with these regulations can be a complex and ongoing process for businesses using AI and synthetic data.
Striking the Balance: A Holistic Approach to Business Analysis
In the age of AI and synthetic data, achieving a balance between benefits and limitations is crucial. To unlock the full potential of these technologies, businesses should consider the following strategies:
Data Governance:
Establish robust data governance practices to ensure data quality, integrity, and compliance with regulations. Regular audits and assessments are essential.
Ethical AI:
Prioritize ethical considerations when developing and deploying AI models. Address biases, and engage in responsible AI practices to avoid unintended consequences.
Skill Development:
Invest in upskilling or hiring professionals with expertise in AI, data science, and data engineering to effectively implement these technologies.
Data Security:
Implement robust data security measures to protect both real and synthetic data. Regularly assess and update these security measures to adapt to evolving threats.
Continuous Learning:
Stay up-to-date with the latest AI and synthetic data trends and best practices. The field is continuously evolving, and businesses that adapt will remain competitive.
EndNote
In conclusion, AI and synthetic data have the potential to revolutionize business analysis by offering automation, insights, and enhanced data privacy. However, their implementation requires a strategic approach that addresses challenges related to data quality, bias, expertise, and compliance. Businesses that strike the right balance between the benefits and limitations of AI and synthetic data will be well-positioned to thrive in the data-driven economy of the future.