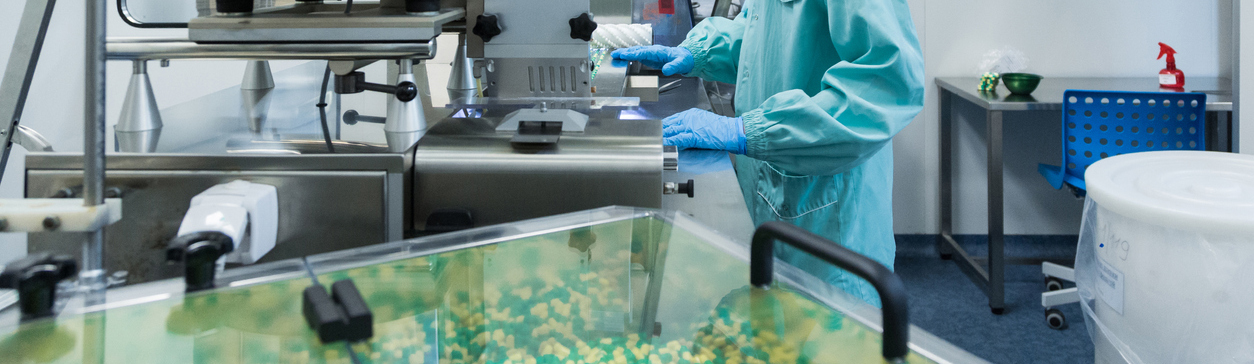
Abstract
In drug manufacturing, keeping track of data is crucial for the drug’s approval from the FDA. The “Continued Process Verification” (CPV) data needs to be maintained for ensuring that the product outputs are within predetermined quality limits. In spite of rising demand for the creation of digital data directly at the source itself, some companies follow the traditional methods of documenting the processes parameters on paper, on designed forms. This leads to data being inaccessible for others unless it is again digitized by someone. The traditional way of achieving this is to have someone enter the data into a computer system by shuffling around the pages in the document. The manual process consumes a lot of time and leaves very little time for the data entered to be validated. This article briefly describes the possible methods of automating the manual data entry process, and how the upcoming technologies can be used for this work.
Introduction
Converting handwritten and typed data from papers into digital formats is one of the most commonly faced challenges across industries today. Keeping data on papers has its own set of limitations like limited accessibility, searchability, using data for analytics, etc.
Digital Transformation of Documents
The digital transformation of such documents is necessary. Over a while, companies have adopted various methods of converting this data into a digital structured format. Some companies hire interns to manually enter the data from the document into excel sheets or word documents, while some companies need the scientists working on the projects to manually enter this data into digital documents. The manual data entry process consumes valuable time and effort from the scientists while explaining the entire process of data entry to new interns consumes time from the team. With advancements in the software industry, there have been multiple attempts to solve these problems, but each solution comes with its own set of limitations.
Robotic Process Automation (RPA)
Robotic Process Automation (RPA) is one of the closest successful solutions in helping companies convert their data from papers to structured digital formats. RPA relies on the rules that the documents might follow. The papers are scanned and processed as an image in the RPA software. The software tries to identify the set of parameters on the image, which need to be translated into the structured database. The set of parameters is searched based on certain rules of the document, which could be the sequence of pages, the sequence of words on the pages, or some other form of a landmark for identifying the parameters on the paper. This approach mostly fails if the paper documents do not follow any template, or there exist multiple pages with similar contents, or if most of the contents on the paper are handwritten. Considering the dynamic nature of the documents, it becomes difficult for the software to define rules based on which the process can be completely automated.
Optical Character Recognition (OCR)
Many solutions/software rely on Optical Character Recognition (OCR) engines as one of the primary components in their toolbox, which is further combined with techniques from the Natural Language Processing (NLP) domain, to try to make sense of the extracted texts. But many solutions fail due to the inability of the OCR to provide accurate results on scanned pages containing hand-written texts, special symbols, marking, notes, etc. This leads to breaking the flow of a possible fully automated solution.
The following sections talk about various possible OCR engines from the leading firms in the market and try to explore and evaluate the performances of the OCRs specifically on hand-written texts. Further, an experiment is performed to try to integrate the OCRs with custom-built software that can use the OCR output and try to structure the data from scanned pages into a database. The pros and cons of this approach are explained in subsequent sections. Furthermore, to overcome the shortfalls of OCR technology, an alternate approach is suggested, using Speech-to-text for data extraction.
Speech-to-text
The speech-to-text approach is also integrated within a custom-built software to evaluate the efficiency of data extraction, in terms of speed and accuracy. The speech-to-text based solution is further investigated on its scalability aspect, and how much time and effort would be needed per batch records are calculated.