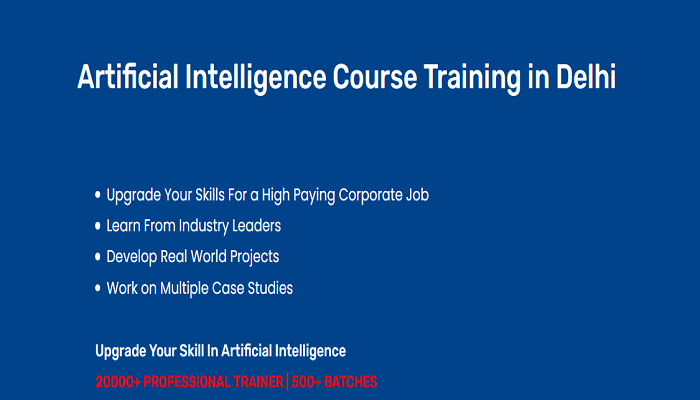
Artificial Intelligence (AI)
Artificial Intelligence uses PCs and machines to copy the critical thinking and dynamic abilities of the human psyche.
What is Artificial Intelligence?
While various meanings of Artificial Intelligence (AI) have surfaced in the course of the most recent couple of many years, John McCarthy offers the accompanying definition in this 2004 paper (PDF, 106 KB) (interface lives outside IBM), " It is the science and designing of making wise machines, particularly savvy PC programs. It is identified with the comparative assignment of utilizing PCs to comprehend human insight, however, AI doesn't need to bind itself to strategies that are organically detectable." Join Artificial Intelligence Course In Delhi.
In any case, many years before this definition, the introduction of the computerized reasoning discussion was signified by Alan Turing's original work, "Figuring Machinery and Intelligence" (PDF, 89.8 KB)(link dwells outside of IBM), which was distributed in 1950. In this paper, Turing, frequently alluded to as the "father of software engineering", poses the accompanying inquiry, "Can machines think?" From there, he offers a test, presently broadly known as the "Turing Test", where a human cross-examiner would attempt to recognize a PC and human content reaction. While this test has gone through much examination since its distribution, it's anything but a significant piece of the historical backdrop of AI just as a continuous idea inside the way of thinking as it uses thoughts around semantics.
Stuart Russell and Peter Norvig then, at that point continued to distribute, Artificial Intelligence: A Modern Approach (connect dwells outside IBM), getting one of the main course books in the investigation of AI. In it, they dive into four likely objectives or meanings of AI, which separates PC frameworks based on discernment and thinking versus acting:
Human methodology:
Frameworks that think like people
Frameworks that behave like people
Optimal methodology:
Frameworks that think soundly
Frameworks that act sanely
Alan Turing's definition would have fallen under the classification of "frameworks that behave like people."
At its least complex structure, man-made consciousness is a field, which joins software engineering and vigorous datasets, to empower critical thinking. It likewise envelops sub-fields of AI and profound realizing, which are much of the time referenced related to computerized reasoning. These orders are contained AI calculations that try to make master frameworks that make expectations or characterizations dependent on input information.
Today, a great deal of publicity actually encompasses AI improvement, which is anticipated from any new arising innovation on the lookout. As verified in Gartner's publicity cycle (connect dwells outside IBM), item advancements like, self-driving vehicles and individual associates, follow "a normal movement of development, from overenthusiasm through a time of bafflement to an inevitable comprehension of the development's importance and job in a market or area." As Lex Fridman notes here (00:15) (interface lives outside IBM) in his MIT address in 2019, we are at the pinnacle of expanded assumptions, moving toward the box of disappointment.
As discussions arise around the morals of AI, we can start to see the underlying looks at the box of frustration. To peruse more on where IBM remains inside the discussion around AI morals, read more here.
Sorts of man-made brainpower—frail AI versus solid AI
Frail AI—additionally called Narrow AI or Artificial Narrow Intelligence (ANI)— is AI prepared and centered to perform explicit assignments. Feeble AI drives the majority of the AI that encompasses us today. 'Tight' may be a more precise descriptor for this kind of AI as it is everything except frail; it empowers some extremely powerful applications, like Apple's Siri, Amazon's Alexa, IBM Watson, and self-sufficient vehicles.
Solid AI is comprised of Artificial General Intelligence (AGI) and Artificial Super Intelligence (ASI). Fake general knowledge (AGI), or general AI, is a hypothetical type of AI where a machine would have an insight approached to people; it's anything but a mindful awareness that can tackle issues, learn, and plan for what's to come. Counterfeit Super Intelligence (ASI)— otherwise called genius—would outperform the insight and capacity of the human mind. While solid AI is still completely hypothetical with no functional models being used today, that doesn't mean AI scientists aren't likewise investigating its turn of events. Meanwhile, the best instances of ASI may be from sci-fi, like HAL, the superhuman, maverick PC partner in 2001: A Space Odyssey.
Profound learning versus AI
Since profound learning and AI will in general be utilized reciprocally, it's significant the subtleties between the two. As referenced above, both profound learning and AI are subfields of man-made consciousness, and profound learning is really a sub-field of AI.
Visual Representation of how AI, ML, and DL identify with each other
Profound learning is really involved neural organizations. "Profound" in profound learning alludes to a neural organization included multiple layers—which would be comprehensive of the sources of info and the yield—which can be viewed as a profound learning calculation. This is by and large addressed utilizing the accompanying chart:
Chart of Deep Neural Network
The manner by which profound learning and AI vary is in how every calculation learns. Profound learning robotizes a significant part of the element extraction piece of the cycle, taking out a portion of the manual human mediation required and empowering the utilization of bigger informational indexes. You can consider profound learning "versatile AI" as Lex Fridman noted in same MIT address from a higher place. Old style, or "non-profound", AI is more subject to human mediation to learn. Human specialists decide the pecking order of highlights to comprehend the contrasts between information inputs, generally requiring more organized information to learn.
"Profound" AI can use named datasets, otherwise called directed learning, to illuminate its calculation, however it doesn't really need a named dataset. It can ingest unstructured information in its crude structure (for example text, pictures), and it can consequently decide the progressive system of highlights that recognize various classes of information from each other. Not at all like AI, it doesn't need human mediation to handle information, permitting us to scale AI in additional fascinating manners.
Computerized reasoning applications
There are various, genuine uses of AI frameworks today. The following are the absolute most normal models:
Discourse acknowledgment: It is otherwise called programmed discourse acknowledgment (ASR), PC discourse acknowledgment, or discourse to-text, and it's anything but an ability that utilizes regular language preparing (NLP) to deal with human discourse into a composed arrangement. Numerous cell phones consolidate discourse acknowledgment into their frameworks to lead voice search—for example Siri—or give greater availability around messaging.
Client support: Online chatbots are supplanting human specialists along the client venture. They answer habitually posed inquiries (FAQs) around subjects, such as transportation, or give customized counsel, strategically pitching items or recommending sizes for clients, changing the manner in which we consider client commitment across sites and online media stages. Models remember informing bots for web based business locales with virtual specialists, informing applications, like Slack and Facebook Messenger, and assignments typically done by remote helpers and voice aides.
PC vision: This AI innovation empowers PCs and frameworks to get significant data from computerized pictures, recordings, and other visual sources of info, and dependent on those data sources, it can make a move. This capacity to give proposals recognizes it from picture acknowledgment errands. Fueled by convolutional neural organizations, PC vision includes applications inside photograph labeling in online media, radiology imaging in medical services, and self-driving vehicles inside the auto business.
Suggestion motors: Using past utilization of conduct information, AI calculations can assist with finding information drifts that can be utilized to foster more viable strategically pitching techniques. This is utilized to make important extra proposals to clients during the checkout interaction for online retailers.
Computerized stock exchanging: Designed to enhance stock portfolios, AI-driven high-recurrence exchanging stages make thousands or even great many exchanges each day without human mediation.
History of man-made brainpower: Key dates and names
The possibility of a machine that thinks' traces all the way back to antiquated Greece. However, since the appearance of electronic processing (and comparative with a portion of the subjects examined in this article) significant occasions and achievements in the development of man-made consciousness incorporate the accompanying: Join Madrid Software for more Information.
1950: Alan Turing distributes Computing Machinery and Intelligence. In the paper, Turing—popular for breaking the Nazi's ENIGMA code during WWII—proposes to respond to the inquiry 'can machine think?' and acquaints the Turing Test with decide whether a PC can show a similar knowledge (or the aftereffects of a similar insight) as a human. The worth of the Turing test has been discussed from that point onward.
1956: John McCarthy coins the term 'man-made consciousness' at the first-historically speaking AI gathering at Dartmouth College. (McCarthy would proceed to develop the Lisp language.) Later that year, Allen Newell, J.C. Shaw, and Herbert Simon make the Logic Theorist, the principal truly running AI programming program.
1967: Frank Rosenblatt assembles the Mark 1 Perceptron, the principal PC dependent on a neural organization that 'learned' through experimentation. Simply a year after the fact, Marvin Minsky and Seymour Papert distribute a book named Perceptrons, which becomes both the milestone work on neural organizations and, basically for some time, a contention against future