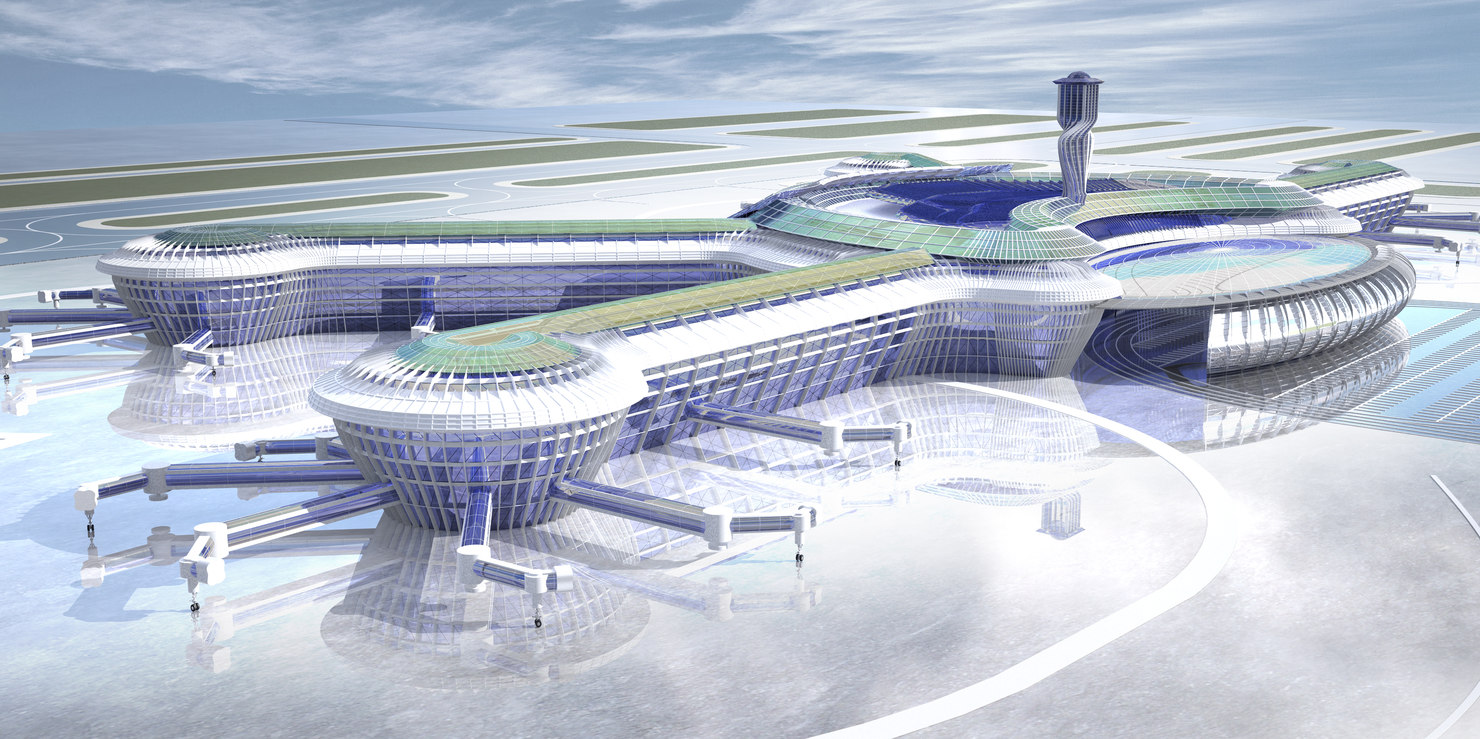
For Unmanned Ground Vehicles (UGVs) equipped with numerous laser scanners, online 3D point cloud categorization and scene interpretation are critical duties. Due to the poor performance of existing 2D image representation models for 3D point clouds, a new Optimal Bearing Angle (OBA) model is presented to address the UGV's on-the-fly navigation restrictions of texture information loss and picture blurring. The centre of points corresponding to each segmented OBA picture patch is allocated as a CRF graph node as a consequence of 3DSLIC-based super-pixel segmentation in OBA images, resulting in a simplified CRF graph structure for online contextual classification of 3D laser pointers in urban contexts. Furthermore, both the raw 3D laser pointers and the related OBA pictures provide a total of 29-dimensional characteristics. In our trials, we employed a significant number of urban scenes from both the DUT2 and KAIST datasets as testing data, and the results demonstrate the validity and performance of the suggested technique. For UGVs functioning in urban areas, 3D laser-based environment sensing and autonomous scene interpretation are tightly tied to semantic mapping and navigation duties. For UGVs to adapt to a range of outdoor environments, a number of laser-based scene understanding algorithms have recently been suggested. Currently, completing the 3D scene interpretation assignment in a real-time framework is a difficult challenge.
Researchers in robotics have lately focused their attention on the topic of autonomous categorization of 3D point clouds. Different types of histogram descriptors were tested for the performance of 3D laser point classification in urban environments in, and it was discovered that 3D histograms based on point distribution, normal orientations, or spectral values performed the best. For urban structure identification, uses an NDT-based laser point classification technique. In addition, a geometric-featured voxel model is used to characterise, segment, and categorise urban buildings. Our new DUT2 dataset was gathered on the Dalian University of Technology campus using a self-developed UGV (see Fig. 1) equipped with two SICK LMS 511 laser range finders facing sideways, similar to the datasets used. The point clouds in our new DUT2 dataset are recorded during theUGV's on-the-fly navigation without pausing regularly for 3D laser scanning, as opposed to the University of Freiburg dataset and our prior DUT1 campus dataset .
Traditional local classifiers perform worse than contextual reasoning approaches like Markov Random Field (MRF) and Conditional Random Field (CRF). To accomplish multi-label 3D point classification, used a functional gradient technique for learning high-dimensional random field parameters. The key contribution of is how higher-order cliques may be used to learn the parameters of a random field. This method was put to the test in a mobile robot moving at 1-2 m/s over various terrains at 1/3Hz for a map of 2550 metres. To improve real-time performance, an SVM-based classifier was trained to perform two-class classification utilising a 2.5D occupancy grid and point feature histograms, with real-time performance exceeding 10Hz. In , a paired CRF technique is employed to achieve semantic 3D point labelling and an OctoMap mapping framework is used to produce 3D Modeling Service are used in semantic outdoor mapping. In addition, to speed up CRF, an adaptively downsampled graph technique based on voxel grids was presented. The processing cost for the point based CRF in cannot fulfil the real-time navigation requirement for UGVs in real-world applications, according to the comparative study of the aforementioned literatures. In contrast to point-based CRF, voxel-grid based CRF in can effectively reduce computational burden, but 3D outdoor scene segmentation results based on voxel-grid model cannot adhere to object's boundaries accurately, resulting in a loss of semantic information and false classification results, particularly in the boundaries of shrub, foliage, car, and lower parts of building facade.
In this research, a new image model known as the Optimal Bearing Angle (OBA) image is suggested for the first time to represent 3D laser points with better performance than the prior Bearing Angle (BA) image. Although the BA picture may be used to do quick scene segmentation and classification and has a lower computational cost than a classifier that uses 3D point clouds directly, the BA model is not appropriate for the 3D laser points acquired in UGV's on-the-fly navigation. Another addition of our research is the CRF graph structure that is built by using superpixel segmentation in OBA pictures instead of 3D point clustering and segmentation. A 29-dimensional feature vector is also designed to complete the parameter training of the CRF-based classification framework (including spatial shape features, directional features, elevation features, statistical point distribution features, and Gray Level Co-occurrence Matrix texture features extracted from OBA image patches). To evaluate the validity and robustness of the suggested technique, a series of experimental findings from both the DUT2 and KAIST datasets are presented.