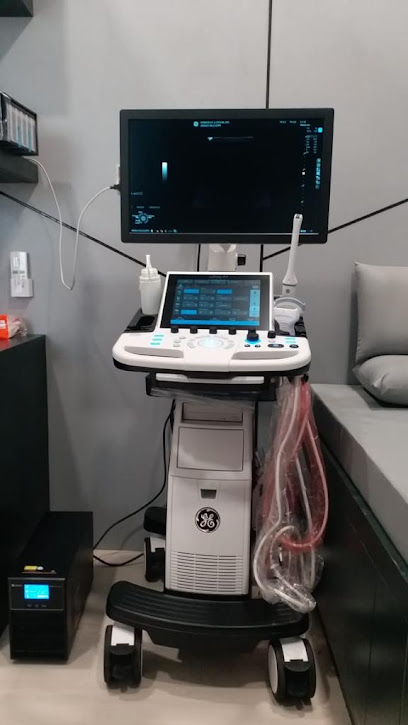
Computed tomography (CT) imaging has broad diagnostic application and is the imaging gold standard for many clinical indications. However, CT imaging exposes patients to higher doses of radiation than other methods. It carries increased cancer risk for all patients, particularly those in higher-risk categories such as pediatric, 2d Echo in Kharghar obese or oncology patients who receive regular screening.
While low-dose and no-dose imaging techniques and modalities exist, a compromise often must be made between patient dose exposure, clinical utility and cost. Within CT, a direct correlation can be drawn between diagnostic image quality, clinical utility and radiation dose exposure. Lower-dose procedures produce noisier images, which can impact clinical utility, radiologist productivity and patient care. Conversely, with increased dose, image quality tends to improve, rendering subtle pathologies more visible — which ultimately benefits radiologists’ diagnostic confidence.
CT imaging protocols can be optimized to adjust dose according to patient and procedure requirements, but this process is complex and cumbersome, resulting in inefficient workflow and increased operational costs. Furthermore, older model CT scanners require higher dose to produce clear images. However, upgrading these devices is often out of reach due to the high associated capital costs. As such, older modalities are often limited to routine cases, resulting in inefficient workload balancing and increased wait times for higher-risk patients.
So, how can healthcare providers balance the requirement for high-quality, precise imaging with tight budgets and the need to reduce radiation exposure risk for their patients? New artificial intelligence-based deep learning reconstruction (DLR) and post-processing techniques have recently become available. These methods can consistently improve diagnostic image quality at the lowest attainable dose across all patients and procedures — far beyond what is possible with current reconstruction techniques. This presents a huge potential for imaging organizations to optimize CT imaging programs.
The Cascading Impact of CT Image Noise
Image “noise” is characterized by unwanted variations in pixel values that cause a grainy or blurry appearance in CT images. Image noise decreases the diagnostic utility of images and reduces the conspicuity of small pathologies. While noise is an inherent part of all CT images, it is more prevalent in lower-dose and thin-slice exams. The paradox between improving diagnostic image quality and reducing dose exposure has a complex and generally negative cascading impact on the clinical and operational aspects of CT workflow.
Poor quality images resulting from high noise are more difficult for radiologists to interpret, forcing them to spend more time carefully reviewing study information. This not only reduces reading efficiency and increases report turnaround time, but it also negatively impacts radiologists’ clinical confidence and the clinical value they can add.
Compounding these challenges is the fact that CT image noise varies across the many CT scanners typically found within a healthcare organization. Because radiologists read studies acquired by these many scanners, they are required to adapt their reading methodology for each. This results in inefficient reading workflow and reduced productivity. It can also be a significant contributor to radiologist frustration and fatigue, due to increased reading burden and constant challenges to their clinical confidence.
The Impact of Iterative Reconstruction
In the late 2000s, iterative reconstruction (IR) was introduced and remains a commonly used technique for improving the quality of CT imaging studies. While IR can reduce image noise and significantly moved the needle in terms of dose reduction, IR images can take substantially longer to process. This approach also has limitations in how much dose can be reduced before images take on a blurry or waxy appearance. IR algorithms are also vendor and scanner specific. This prevents organizations from upgrading all their CT scanners to IR technologies without sweeping and costly capital equipment replacements. The result has been a phased-in adoption of IR over many years, as CT systems are upgraded or replaced.
Images processed by IR have a different appearance and can introduce distinct artifacts and textures that radiologists have had to become familiar with before they can be confident in their clinical diagnoses. While the promise of IR has been largely realized, the limitations of IR cannot be ignored. We have now entered the next phase of CT image reconstruction and post-processing where AI technologies will help to overcome these limitations and push CT imaging into another era.