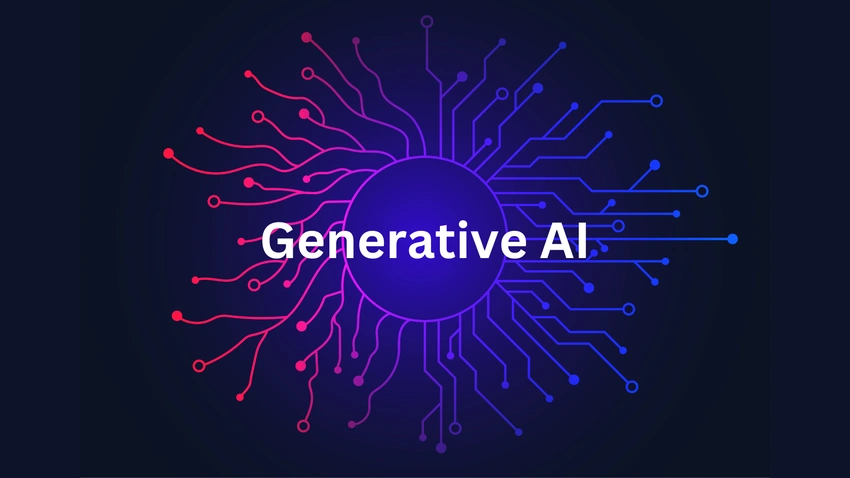
The landscape of artificial intelligence has witnessed numerous cycles of excitement, yet the introduction of ChatGPT appears to signal a pivotal moment, even for skeptics. OpenAI's chatbot, fueled by its cutting-edge large language model, showcases the ability to craft poems, jokes, and essays that convincingly emulate human creation. A mere prompt to ChatGPT can result in love poems masquerading as Yelp reviews or song lyrics echoing the style of Nick Cave.
In the past, generative AI took center stage with breakthroughs in computer vision, transforming selfies into Renaissance-style portraits and aging faces prematurely on social media. However, the current surge in natural language processing, specifically the prowess of large language models to creatively engage with diverse themes, has captivated popular imagination. Beyond language, generative models demonstrate proficiency in learning the grammar of software code, molecules, natural images, and various other data types.
The applications of this technology are expanding daily, ushering us into an era of exploration for its potential. At IBM Research, efforts are underway to empower customers in using generative models for accelerated software code development, the discovery of new molecules, and the training of reliable conversational chatbots grounded in enterprise data. Additionally, generative AI is being leveraged to generate synthetic data, addressing the need for robust and trustworthy AI models while respecting privacy and copyright laws.
As the field progresses, it becomes essential to revisit the fundamentals of generative AI, its evolution, and operational principles.
Understanding Generative AI Models
Generative AI refers to deep-learning models capable of taking raw data, such as the entirety of Wikipedia or the works of Rembrandt, and learning to generate statistically probable outputs in response to prompts. These models encode a simplified representation of their training data and draw from it to produce new content that shares similarities with, but is not identical to, the original data.
The ascent of deep generative models traces back to variational autoencoders (VAEs), introduced in 2013. VAEs, by making models easier to scale, played a pivotal role in extending generative models beyond numerical data to encompass images and speech. Autoencoders, the foundation of VAEs, encode unlabeled data into a compressed form and then decode it back into its original structure. Variational autoencoders introduced the crucial ability to output variations on the original data, paving the way for subsequent models like generative adversarial networks (GANs) and diffusion models, capable of producing realistic yet synthetic content.
Transformers, introduced by Google in 2017, marked another milestone. Combining an encoder-decoder architecture with attention mechanisms, transformers revolutionized how language models were trained. These models, known as foundation models, could be pre-trained on vast amounts of raw text and later fine-tuned for specific tasks with minimal labeled data. The versatility of transformers is evident in their applications for both non-generative tasks like classification and generative tasks such as translation, summarization, and question answering.
Evolution of Generative AI
The progression of generative AI has been marked by a shift towards larger models, exemplified by Google's GPT-3. These models, with their massive parameters, are capable of generating convincing dialogue, essays, and various content types. Language transformers fall into three categories: encoder-only models (e.g., BERT), decoder-only models (e.g., GPT-3), and encoder-decoder models (e.g., Google's Text-to-Text Transfer Transformer, T5).
While the ability to leverage unlabeled data was a crucial innovation, human supervision has staged a comeback. Supervised learning, particularly instruction-tuning, allows generative models to move beyond simple tasks and offer interactive, generalized assistance. The use of prompts, carefully engineered initial inputs, enables customization of models for a wide range of tasks, with the advent of zero-shot and few-shot learning reducing the need for extensive labeled data.
The Role of Human Supervision
Human supervision is shaping generative models by aligning their behavior with human expectations. Reinforcement learning from human feedback (RLHF) has gained prominence, as exemplified by OpenAI's approach in training models like ChatGPT. In RLHF, models output candidate responses rated by humans for correctness, adjusting the model through reinforcement learning to produce high-quality conversational text aligned with human preferences.
The Future of Generative AI
The trajectory of generative AI poses intriguing questions about the scale of models. While there has been a trend towards larger models, recent evidence suggests that smaller, domain-specialized models trained on specific datasets can outperform larger, general-purpose counterparts. The emergence of model distillation, where capabilities of large models are infused into smaller ones, challenges the necessity of massive models for certain applications.
Generative AI holds immense potential for enterprise applications but introduces new challenges, including legal, financial, and reputational risks. Addressing issues like hallucinations, biases, and privacy concerns will be crucial as generative models become integral to diverse industries. As we navigate the evolving landscape of generative AI, a balance between model scale, specialization, and ethical considerations will shape its trajectory.