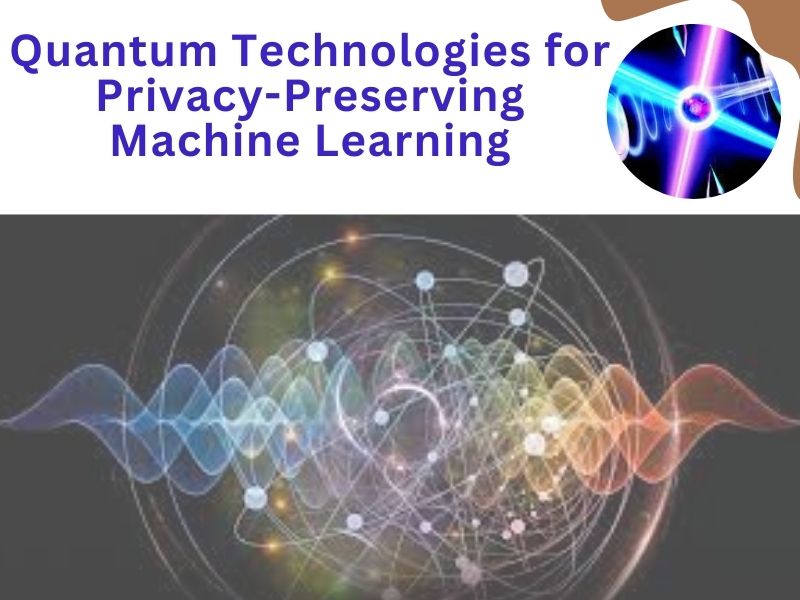
Quantum technologies hold significant promise in the realm of privacy-preserving machine learning, providing innovative solutions to enhance data security and confidentiality. Privacy concerns are paramount in the era of big data, and quantum technologies offer unique capabilities to protect sensitive information during machine learning tasks. In this context, we'll explore key quantum technologies contributing to privacy-preserving machine learning.
Quantum Key Distribution (QKD)
Quantum Key Distribution is a revolutionary quantum technology that enables secure communication by leveraging the principles of quantum mechanics to distribute cryptographic keys. In privacy-preserving machine learning, QKD can be used to establish secure communication channels, ensuring that sensitive data remains confidential during transmission.
Quantum Homomorphic Encryption
Homomorphic encryption allows computations to be performed on encrypted data without decrypting it. Quantum homomorphic encryption extends this concept to the quantum domain, enabling secure computation on quantum-encrypted data. This technology is particularly relevant for privacy-preserving machine learning tasks where data privacy is of utmost importance.
Quantum Secure Multi-Party Computation (SMPC)
Quantum Secure Multi-Party Computation involves multiple parties collaboratively computing a function over their inputs while keeping those inputs private. Quantum SMPC leverages the principles of quantum entanglement and superposition to enhance the security of multi-party computations, making it suitable for privacy-preserving machine learning scenarios.
Quantum Randomness
Quantum technologies provide a new level of randomness that can enhance the security of cryptographic protocols. Quantum random number generators can be employed in privacy-preserving machine learning to generate secure keys and masks, adding an additional layer of protection against adversarial attacks.
Click Here, To Enroll In Data Science Course with 100% Guarantee.
Quantum-Safe Cryptography
As quantum computers threaten the security of classical cryptographic algorithms, the development of quantum-safe cryptographic primitives becomes crucial. Quantum-safe cryptography ensures that machine learning models and data remain secure even in the advent of powerful quantum computers capable of breaking current encryption schemes.
Quantum Neural Networks (QNNs)
Quantum neural networks leverage quantum computing principles to perform machine learning tasks. In the context of privacy preservation, quantum neural networks can process encrypted data directly, mitigating the need for data decryption during training and inference, thus preserving the confidentiality of sensitive information.
Quantum Zero-Knowledge Proofs
Zero-knowledge proofs allow one party to prove the knowledge of certain information without revealing the information itself. Quantum zero-knowledge proofs leverage quantum technologies to enhance the security and efficiency of these proofs, ensuring that privacy-preserving machine learning protocols are robust against malicious actors.
Quantum-Secure Blockchain
Blockchain technology, when combined with quantum-safe cryptographic algorithms, provides a secure and transparent platform for privacy-preserving machine learning. Quantum-resistant blockchain ensures the integrity and confidentiality of data stored on the blockchain, facilitating secure collaborative learning scenarios.
Quantum Secure Authentication
Quantum technologies can enhance the security of authentication protocols, protecting access to machine learning models and data. Quantum-resistant authentication mechanisms provide a robust defense against unauthorized access and identity theft in privacy-preserving machine learning environments.
Quantum-Safe Federated Learning
Federated learning enables model training across decentralized devices without sharing raw data. Quantum-safe federated learning leverages quantum technologies to enhance the security of model updates and aggregation, ensuring that privacy is maintained across distributed nodes.
In conclusion, quantum technologies offer a suite of tools and techniques to address privacy concerns in machine learning applications. From secure communication using Quantum Key Distribution to quantum homomorphic encryption and quantum neural networks, these advancements contribute to the development of privacy-preserving machine learning protocols. As quantum technologies continue to evolve, they are poised to play a crucial role in shaping the future of secure and privacy-aware machine learning ecosystems.
banking and investment courses
investment banking certification