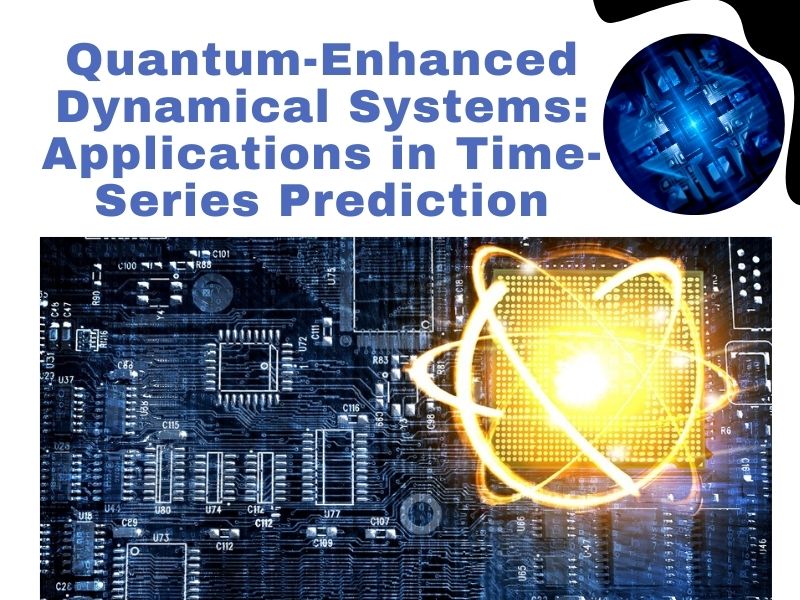
Quantum-enhanced dynamical systems, leveraging the principles of quantum mechanics to improve the modeling and prediction of time-series data, represent a frontier in quantum computing applications. The unique features of quantum computers, such as superposition and entanglement, offer potential advantages in solving complex problems in time-series prediction. Here, we explore the applications of quantum-enhanced dynamical systems in time-series prediction:
Quantum Machine Learning Algorithms
Quantum machine learning algorithms, including quantum support vector machines, quantum neural networks, and quantum-enhanced classifiers, can be applied to time-series prediction tasks. Quantum algorithms may offer speedups over classical counterparts in processing large volumes of time-series data and identifying complex patterns.
Quantum Fourier Transform for Signal Analysis
The Quantum Fourier Transform (QFT) is a quantum algorithm that efficiently analyzes the frequency components of time-series data. Quantum-enhanced Fourier analysis can provide advantages in identifying periodic patterns and extracting relevant features, contributing to more accurate time-series predictions.
Quantum State Estimation
Quantum state estimation techniques can be applied to estimate the underlying states of a dynamical system from observed time-series data. Quantum algorithms can enhance the precision of state estimation, leading to improved predictions of future states in time-series sequences.
Quantum Reservoir Computing
Reservoir computing is a machine learning paradigm for time-series prediction. Quantum-enhanced reservoir computing utilizes quantum devices to perform computations, potentially offering advantages in terms of computational power and the ability to handle intricate temporal dependencies in time-series data.
Quantum-Assisted Bayesian Inference
Bayesian inference is a powerful tool for updating predictions based on new observations. Quantum algorithms for Bayesian inference can enhance the efficiency of updating probability distributions and making predictions in dynamic time-series environments.
Quantum Walks for Temporal Dynamics
Quantum walks, a quantum analog of classical random walks, can be employed for modeling temporal dynamics in time-series prediction. Quantum-enhanced temporal modeling may capture intricate patterns and dependencies in time-series data more effectively than classical approaches.
Click Here, To Enroll in Data Science Course With 100% Placement Guarantee
Quantum Autoencoders for Feature Learning
Quantum autoencoders leverage quantum circuits to efficiently learn compact representations of time-series data. By capturing essential features in a quantum state, these models contribute to more efficient feature learning and improved predictive performance.
Quantum Principal Component Analysis (PCA)
Quantum PCA algorithms can extract principal components from time-series data, reducing dimensionality while retaining essential information. Quantum-enhanced PCA may contribute to more efficient representation and prediction of underlying patterns in time-series sequences.
Quantum Support Vector Machines (SVM)
Quantum support vector machines can be applied to time-series classification and regression tasks. Quantum-enhanced SVMs may offer advantages in handling non-linearities and complex relationships in time-series data, leading to more accurate predictions.
Quantum Ensemble Methods
Quantum ensemble methods, where multiple quantum models are combined to make predictions, can enhance the robustness and accuracy of time-series predictions. Quantum-enhanced ensemble methods leverage the strengths of diverse quantum models to improve overall predictive performance.
Quantum Transfer Learning
Quantum transfer learning involves leveraging knowledge from a source time-series domain to enhance predictions in a target domain. Quantum algorithms for transfer learning can contribute to more efficient adaptation of predictive models across related time-series tasks.
Quantum Error Correction for Robustness
Quantum error correction techniques are crucial for ensuring the robustness of quantum-enhanced dynamical systems. By mitigating the impact of errors in quantum computations, error correction contributes to the reliability and accuracy of time-series predictions.
Conclusion
Quantum-enhanced dynamical systems show promising applications in improving the accuracy and efficiency of time-series prediction tasks. As quantum computing technologies continue to advance, these applications have the potential to revolutionize the field of time-series analysis, offering new insights and capabilities for predicting complex temporal behaviors in diverse domains.
data science course in chennai
data analyst course in chennai
data analytics courses chennai
data analysis course in chennai