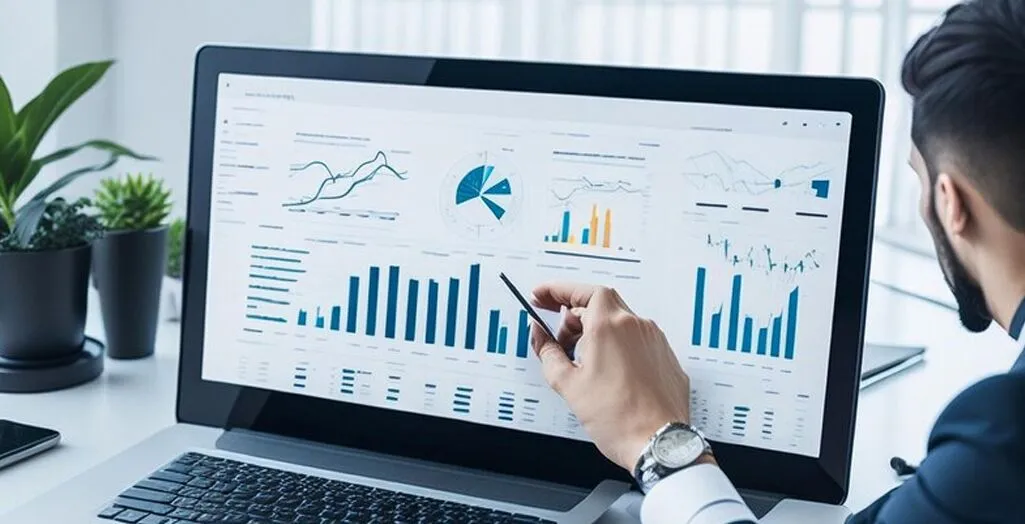
Big data analytics sounds like a magic tool for businesses, promising to turn heaps of data into valuable insights.
But hold on — it’s not all smooth sailing! Just like any big adventure, there are challenges waiting to be tackled. Think of it as a treasure hunt where you have to overcome obstacles to find the gold.
So, before diving headfirst into the world of big data analytics, let’s take a closer look at these challenges and how to conquer them.
1. Data Quality and Integrity
Ensuring the quality and integrity of data is paramount for accurate analysis and decision-making. Big datasets often contain noise, inconsistencies, and inaccuracies that can skew results and undermine the reliability of insights. Data cleansing and validation processes are essential to identify and rectify errors, anomalies, and missing values, but they can be time-consuming and resource-intensive.
2. Data Privacy and Security
As organizations collect, store, and analyze increasingly large volumes of data, ensuring data privacy and security becomes a critical concern. Protecting sensitive customer information, intellectual property, and proprietary data from unauthorized access, breaches, and cyberattacks requires robust security measures, encryption techniques, and compliance with regulatory standards such as GDPR and CCPA.
3. Scalability and Infrastructure
Scaling Big Data Analytics infrastructure to accommodate growing volumes of data and increasing computational requirements can be challenging. Traditional IT architectures may struggle to handle the massive scale and complexity of Big Data Analytics workloads, necessitating investments in scalable storage solutions, distributed computing frameworks, and cloud-based platforms.
4. Talent and Skills Gap
The demand for skilled data scientists, analysts, and engineers proficient in Big Data Analytics tools and techniques often outpaces the supply. Recruiting and retaining top talent with expertise in data management, statistical analysis, machine learning, and programming languages such as Python and R can be a significant challenge for organizations looking to build and sustain effective Big Data Analytics teams.
5. Integration and Interoperability
Integrating disparate data sources, systems, and applications to create a unified data ecosystem is a complex and ongoing process. Achieving seamless interoperability between legacy systems, cloud-based platforms, and third-party applications requires careful planning, data governance, and API integration strategies to ensure data consistency, reliability, and accessibility across the organization.
6. Cost and ROI
Investing in Big Data Analytics initiatives involves substantial upfront costs for infrastructure, software licenses, and talent acquisition. Calculating and demonstrating return on investment (ROI) can be challenging, particularly for long-term projects with uncertain outcomes.
Want to learn more about these challenges and how to tackle them? Check out the full blog here!